
Create
Create
Create
Create
Trustworthy AI
Trustworthy AI
Trustworthy AI
Trustworthy AI
World’s first comprehensive evaluation and optimization platform to help enterprises achieve 99% accuracy in AI applications across software and hardware.
World’s first comprehensive evaluation and optimization platform to help enterprises achieve 99% accuracy in AI applications across software and hardware.
World’s first comprehensive evaluation and optimization platform to help enterprises achieve 99% accuracy in AI applications across software and hardware.

Create
Trustworthy AI
World’s first comprehensive evaluation and optimization platform to help enterprises achieve 99% accuracy in AI applications across software and hardware.
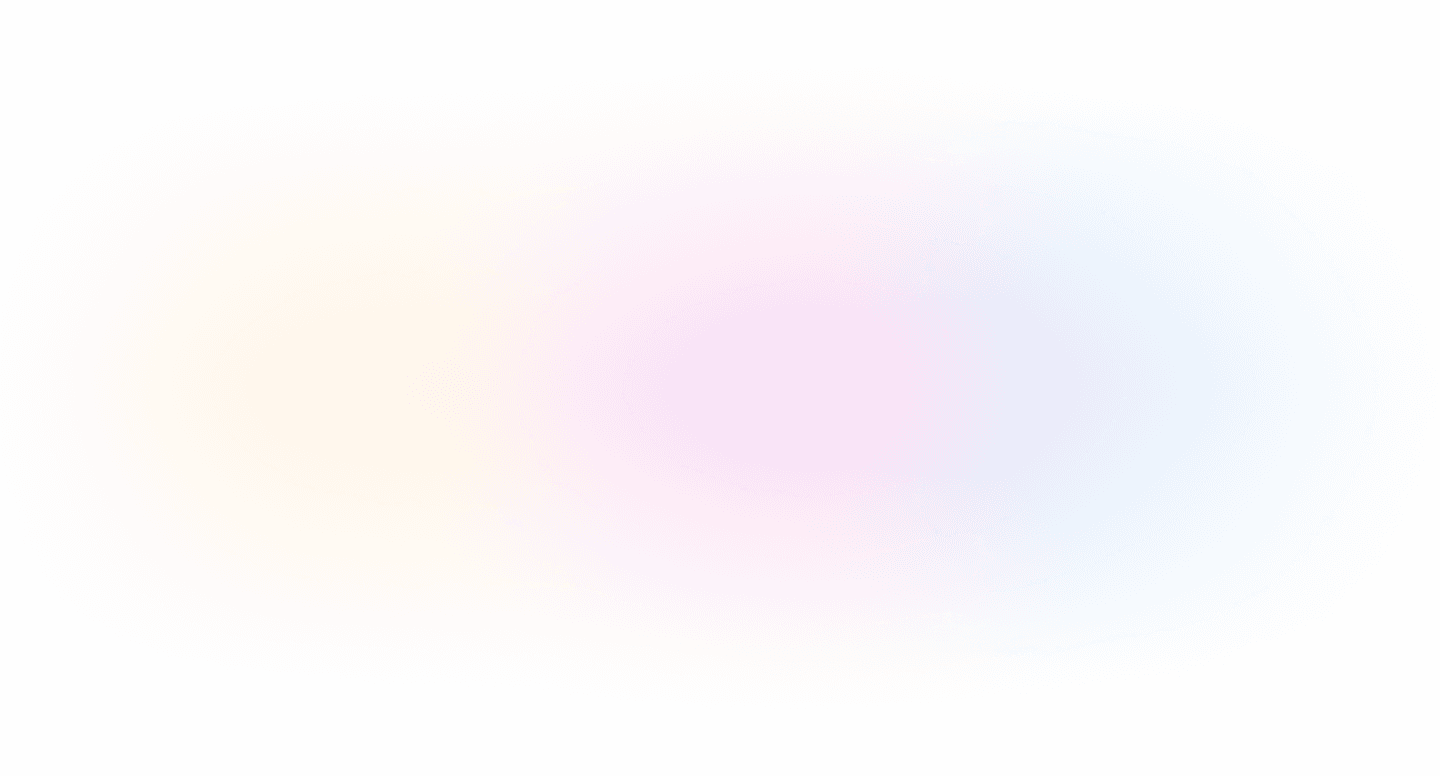
Integrated with
Integrated with
Faster AI Evaluation
Faster Agent Optimization
Model and Agent Accuracy in Production
Faster AI Evaluation
Faster Agent Optimization
Model and Agent Accuracy in Production
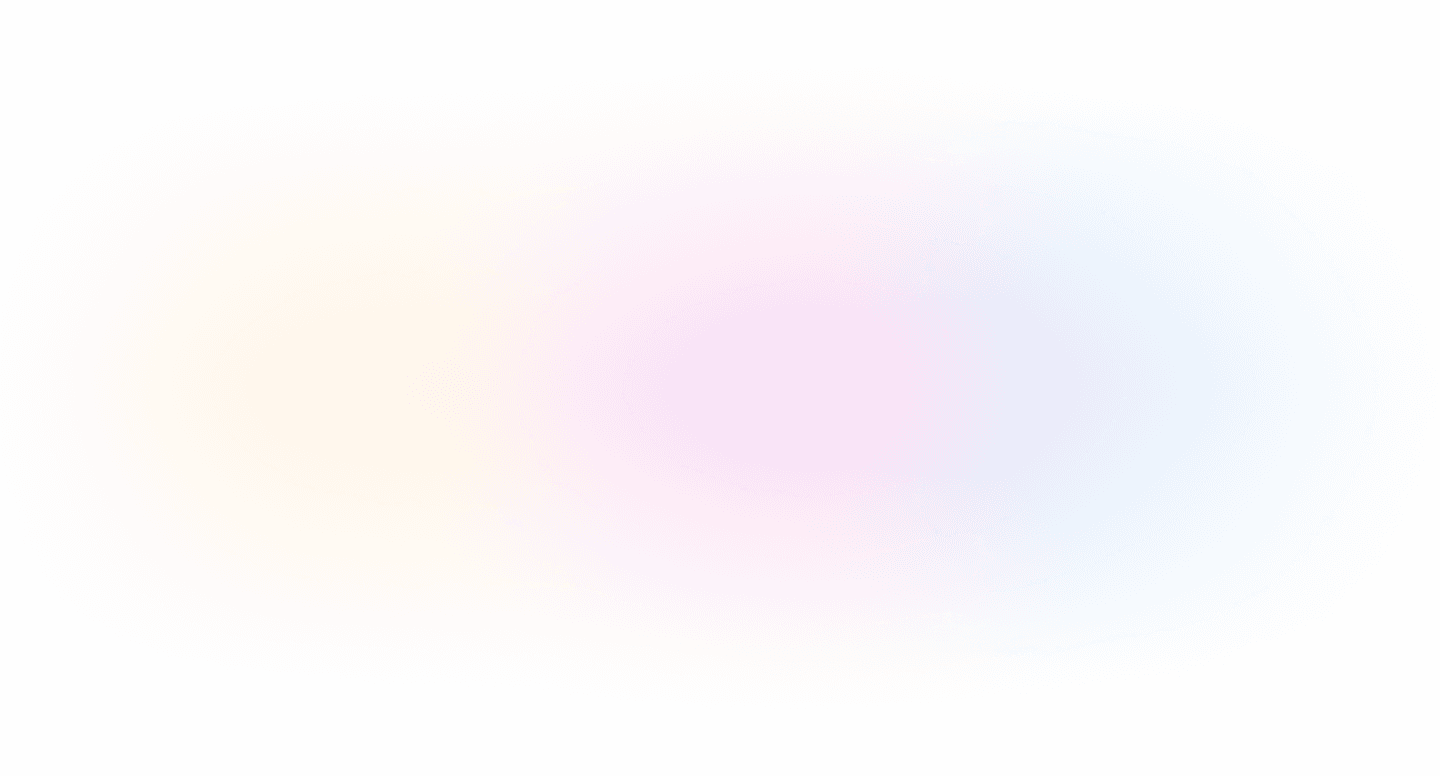
Integrated with
Faster AI Evaluation
Faster Agent Optimization
Model and Agent Accuracy in Production
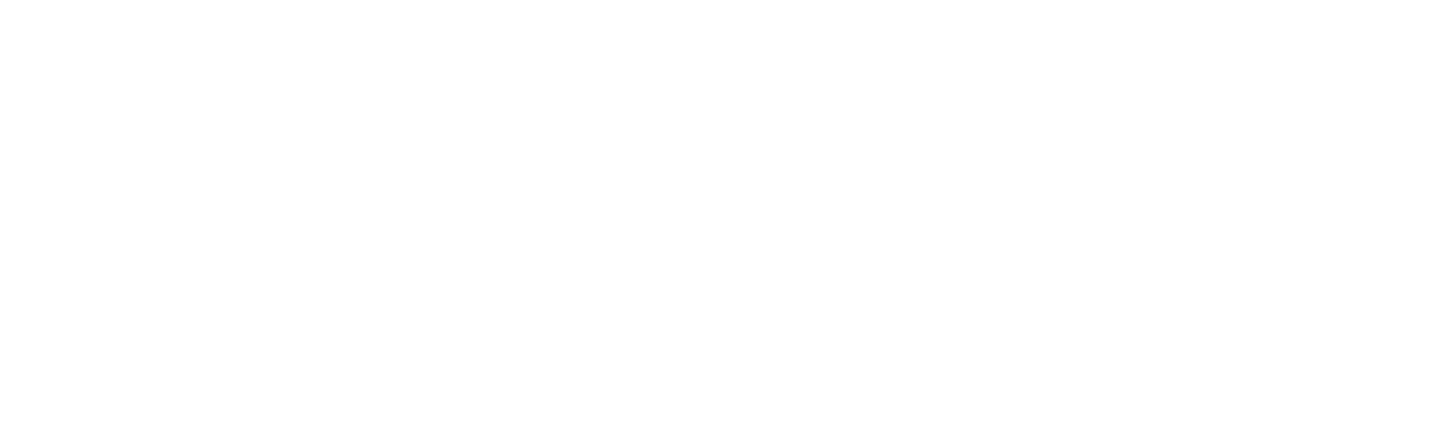
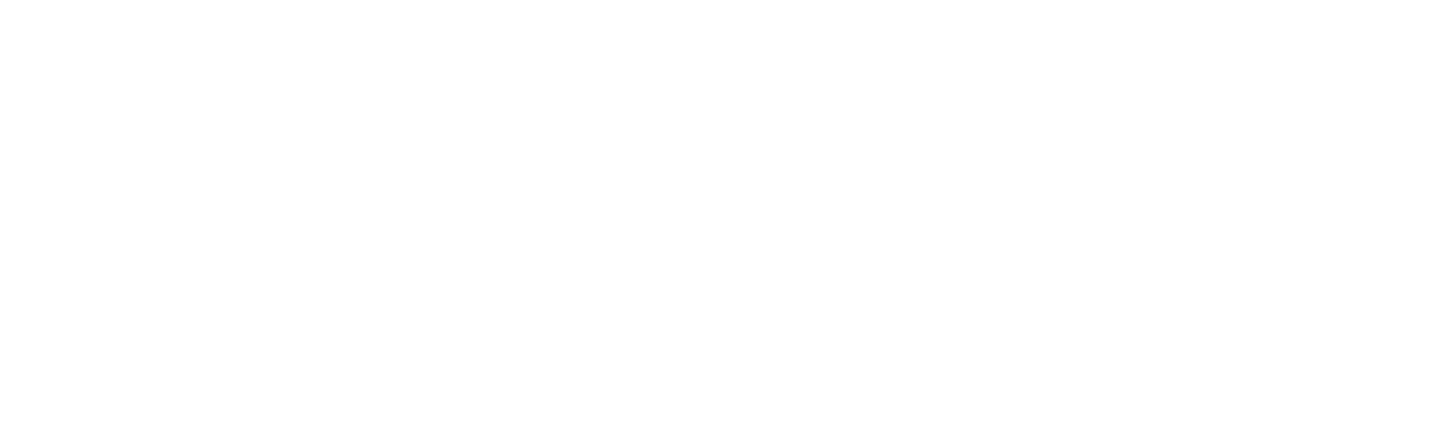
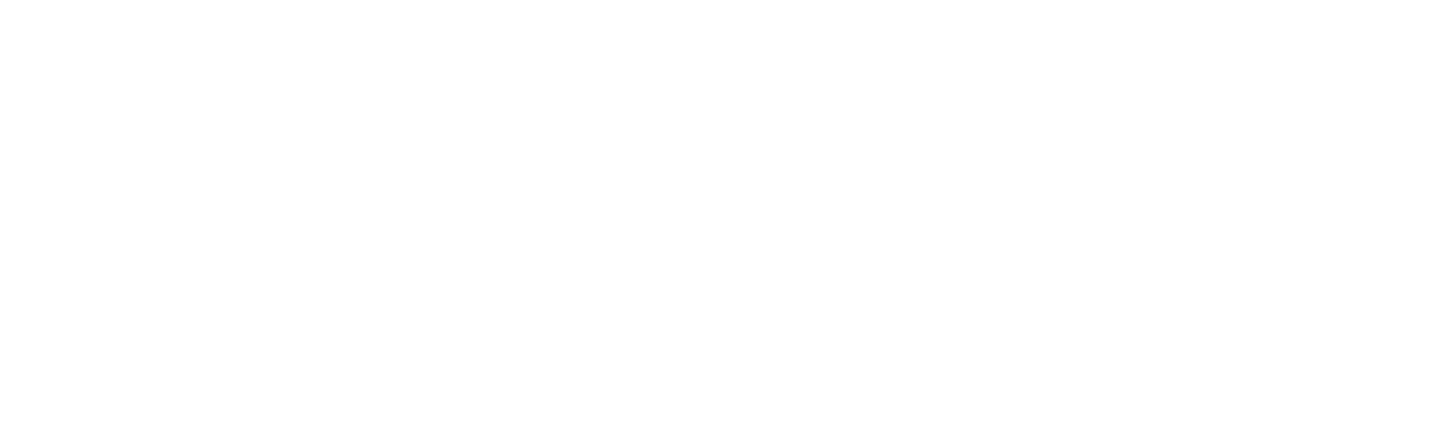
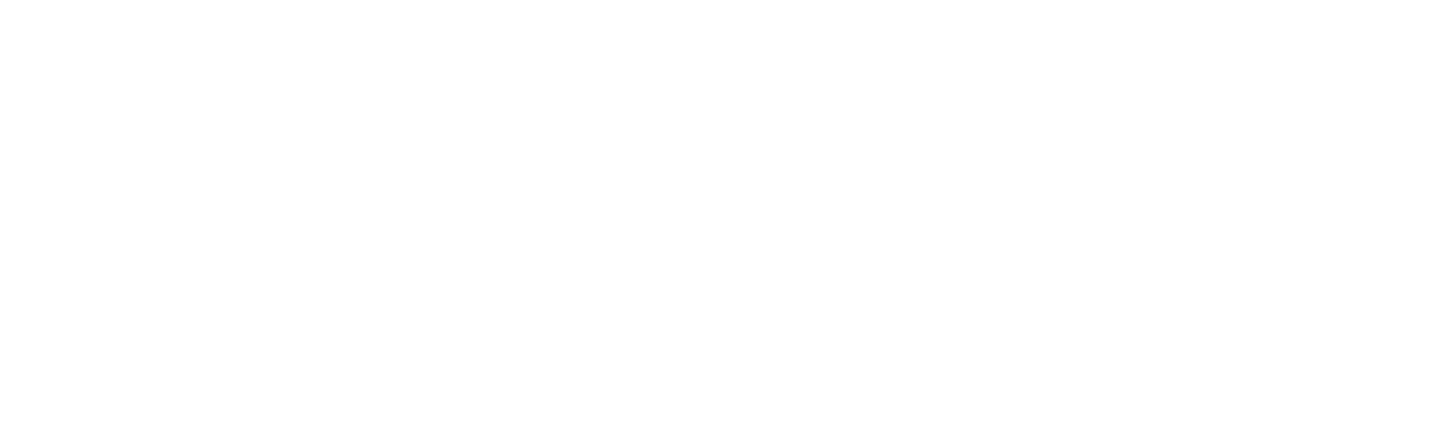
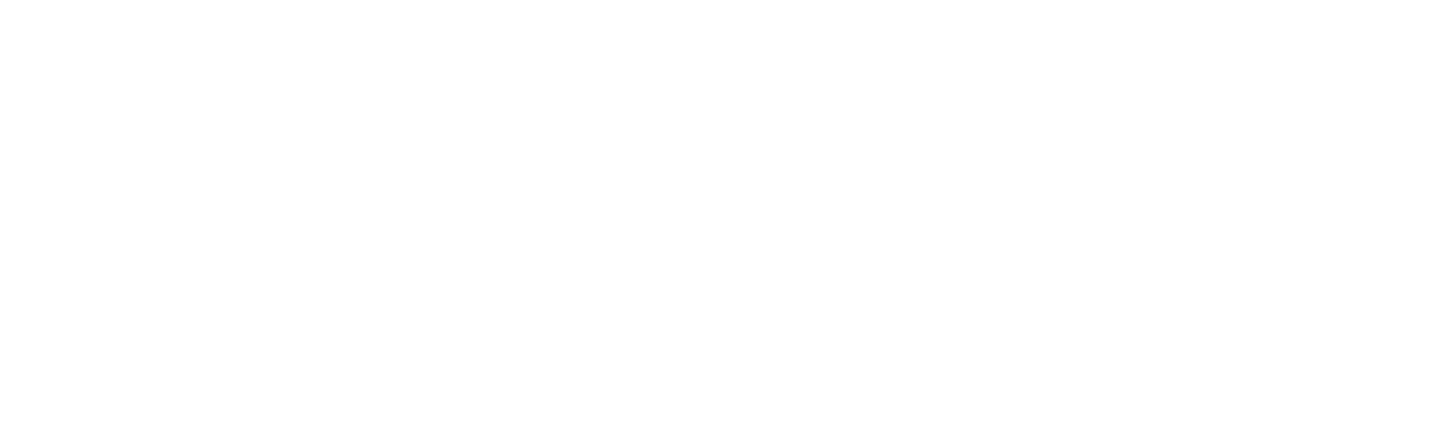
LLMs are probabilistic.
Build, Evaluate and Improve AI reliably with Future AGI.
Build, Evaluate and Improve AI reliably with Future AGI.
Build, Evaluate and Improve AI reliably with Future AGI.
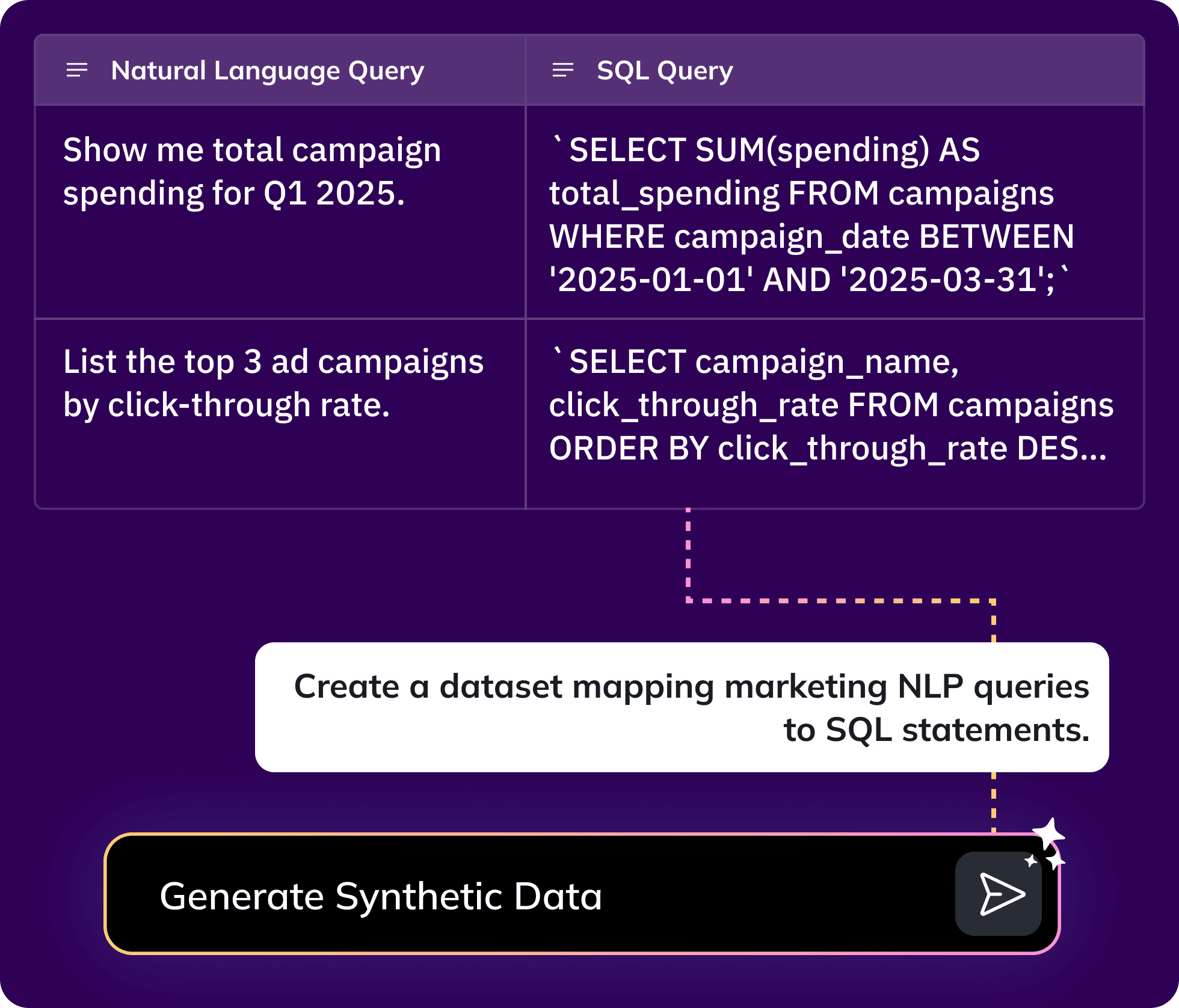
Generate and manage diverse synthetic datasets to effectively train and test AI models, including edge cases.
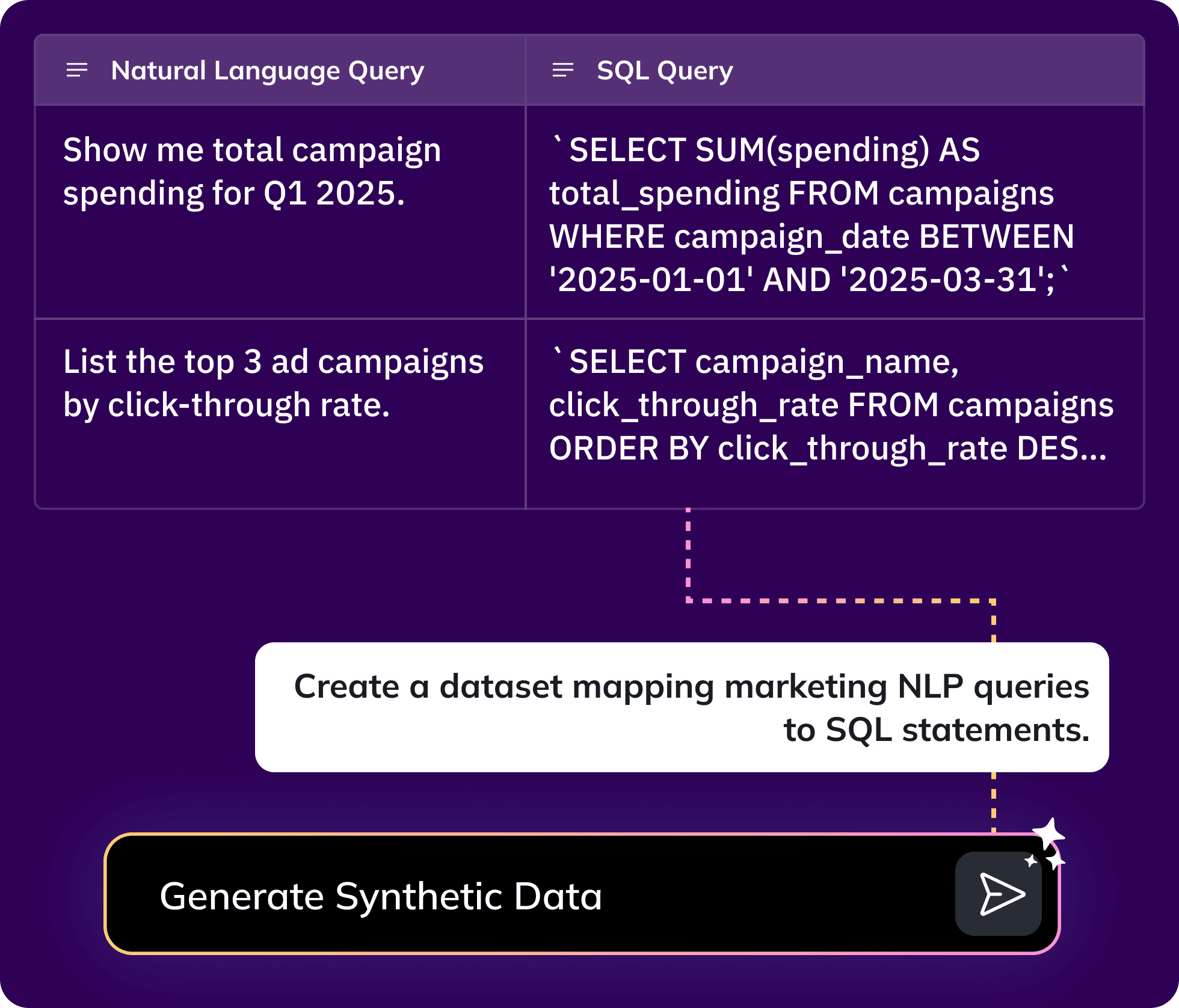
Generate and manage diverse synthetic datasets to effectively train and test AI models, including edge cases.
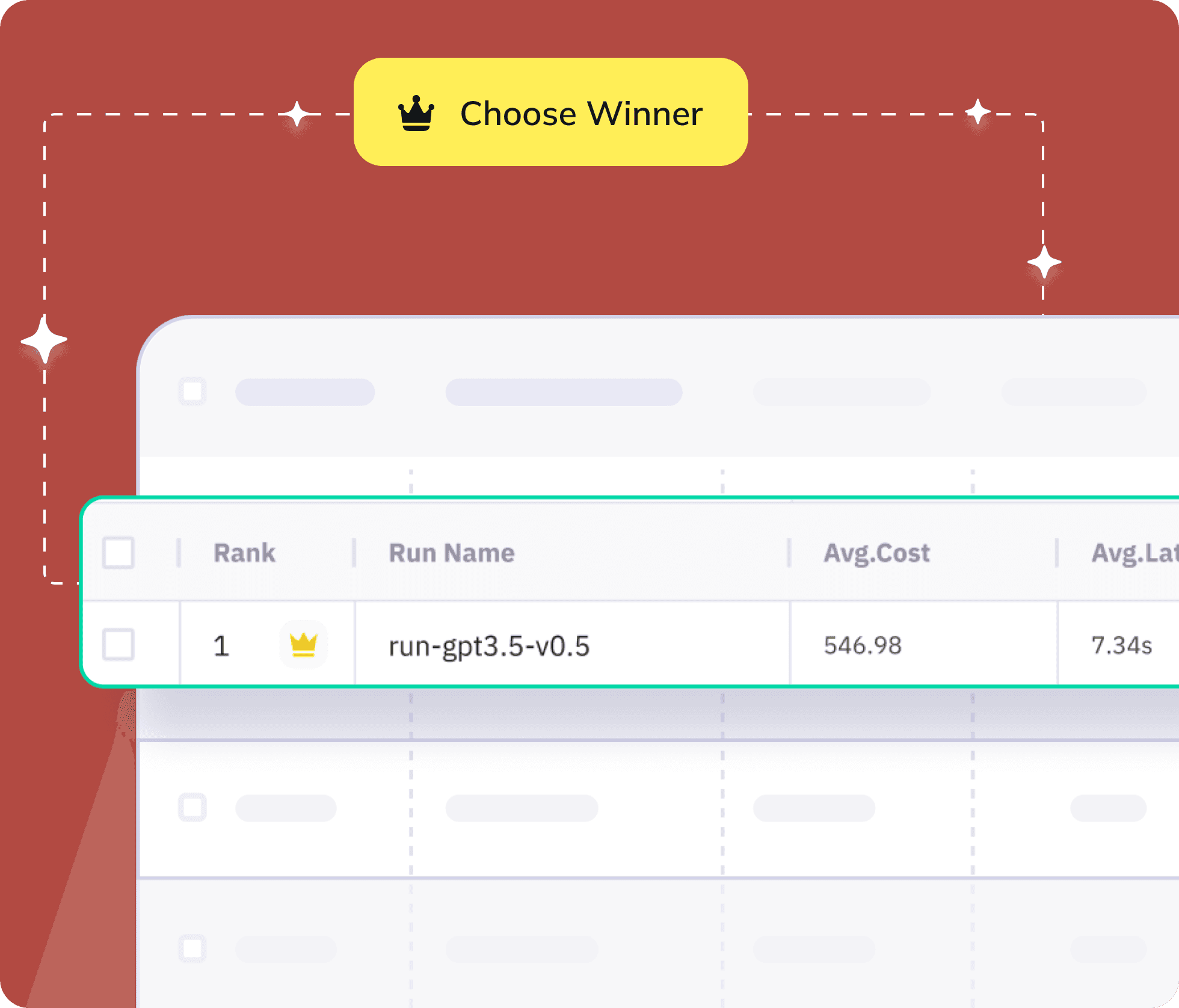
Test, compare and analyse multiple agentic workflow configurations to identify the ‘Winner’ based on built-in or custom evaluation metrics- literally no code!
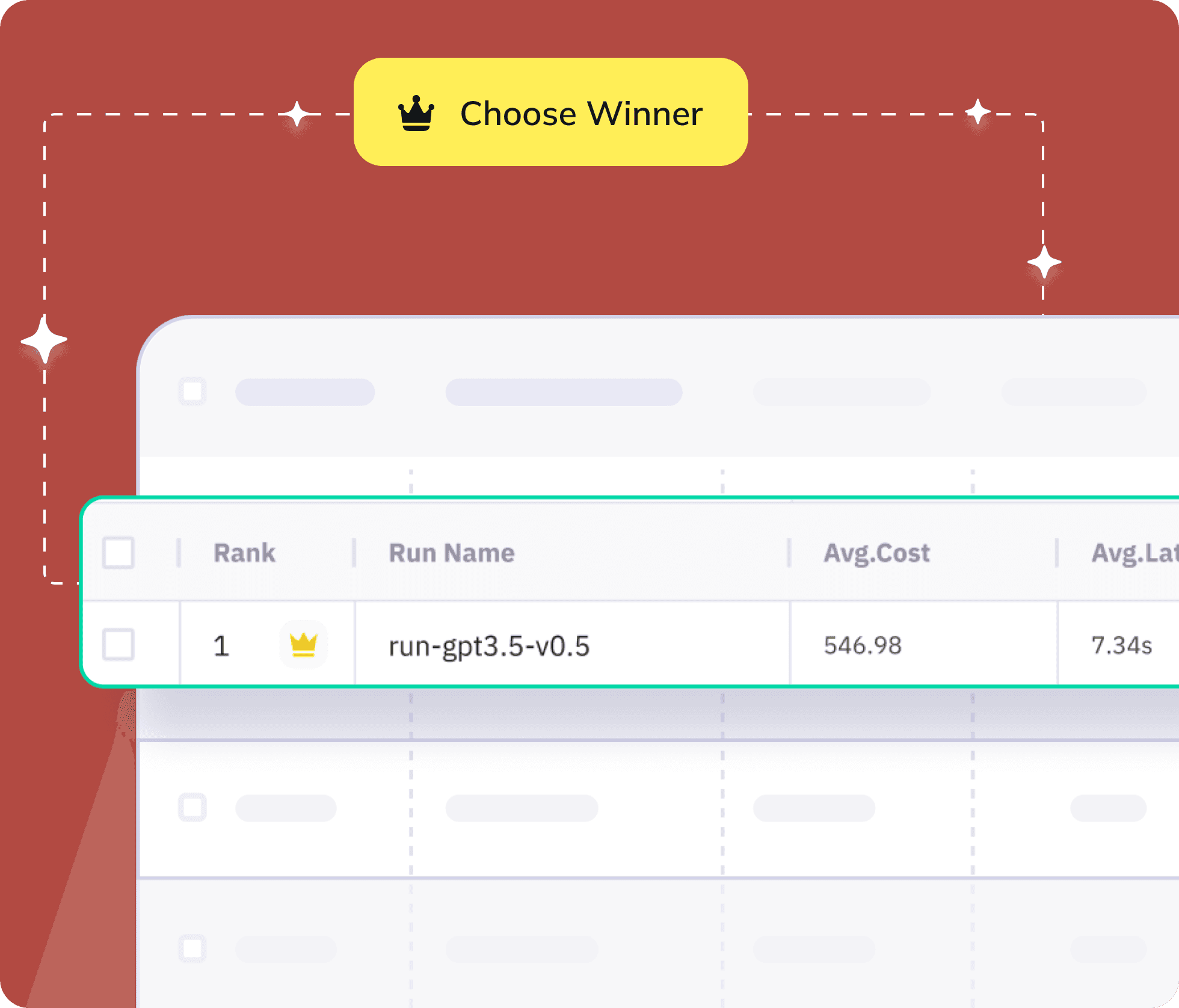
Test, compare and analyse multiple agentic workflow configurations to identify the ‘Winner’ based on built-in or custom evaluation metrics- literally no code!
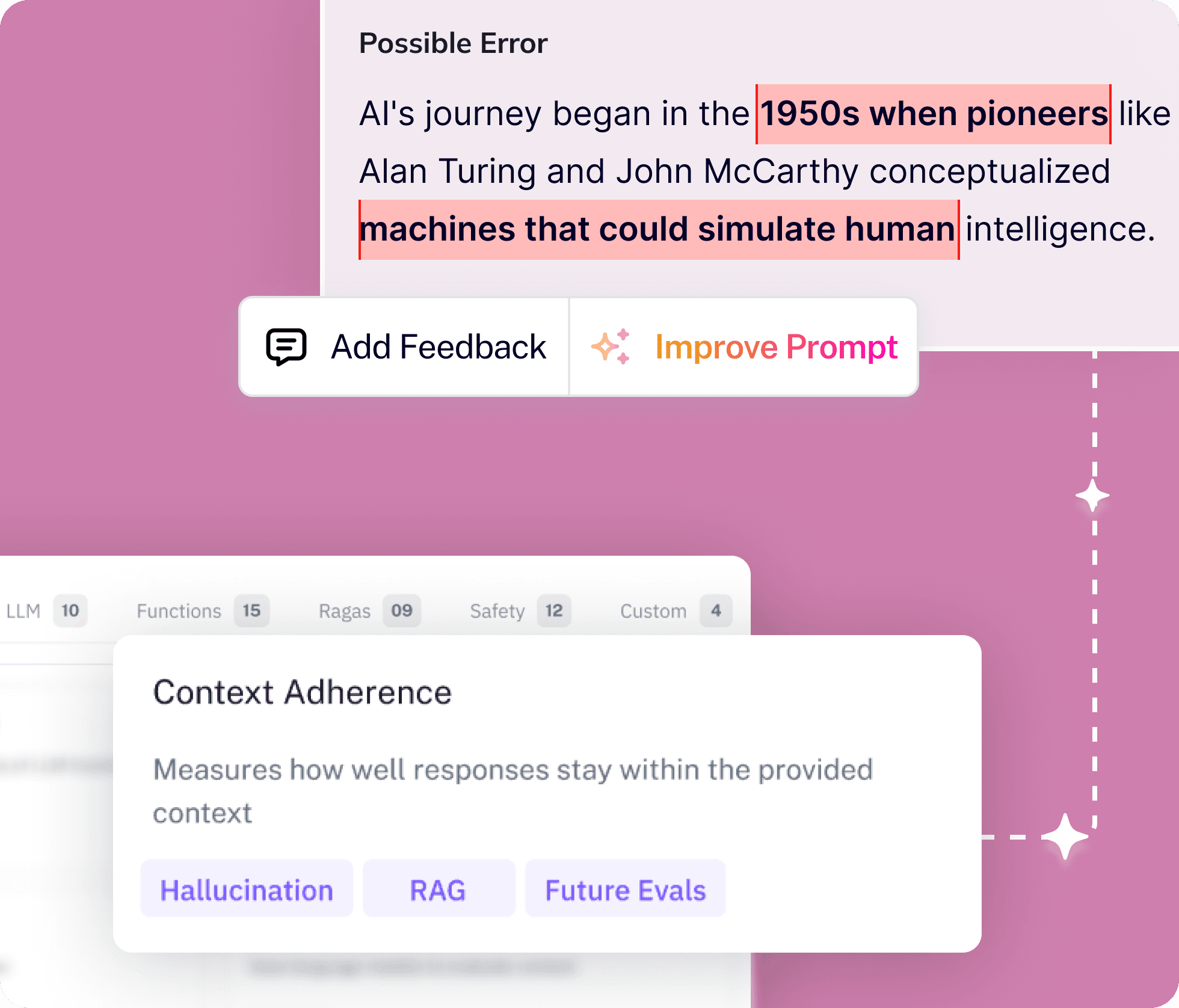
Assess and measure agent performance, pin-point root cause and close loop with actionable feedback using our proprietary eval metrics.
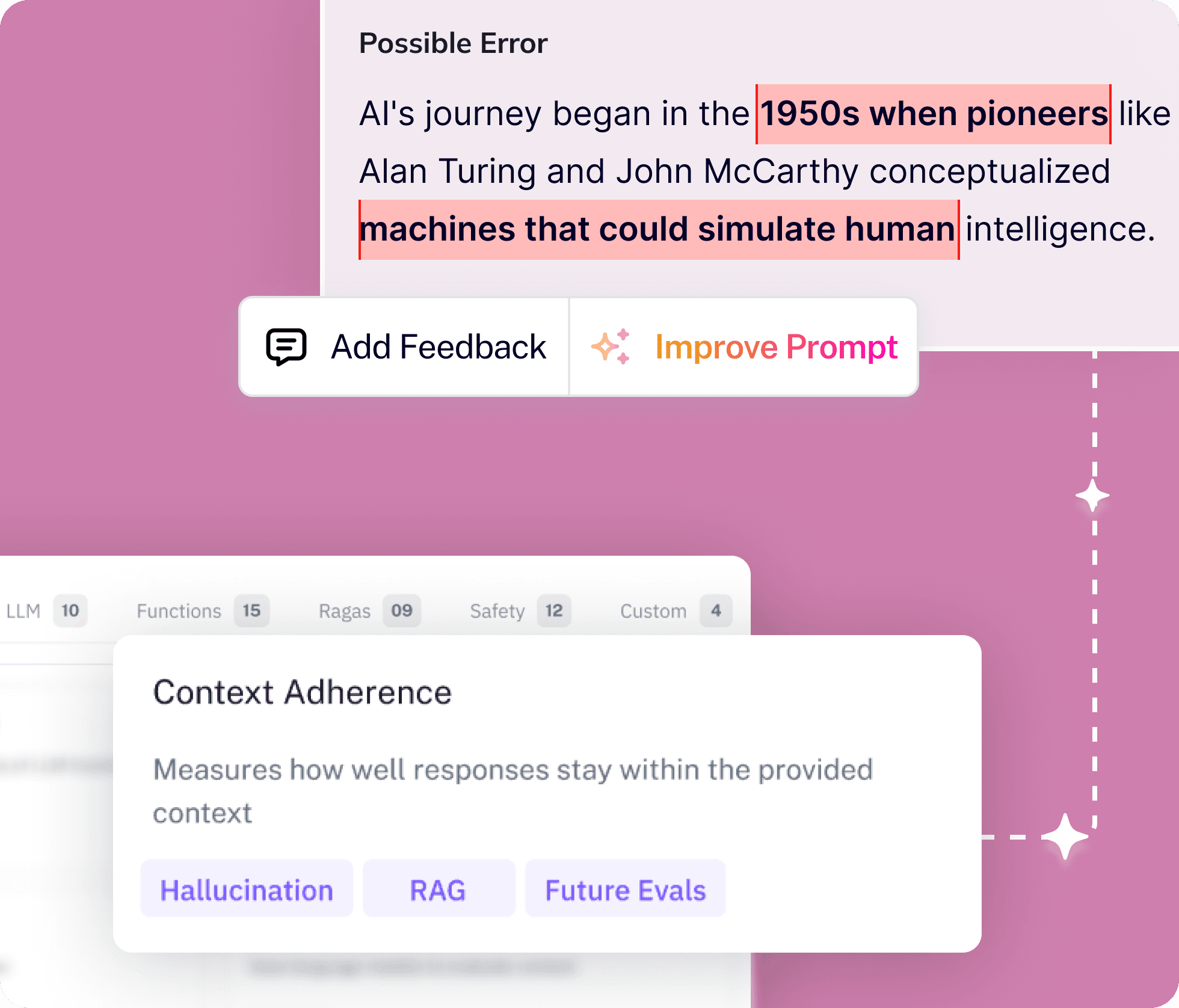
Assess and measure agent performance, pin-point root cause and close loop with actionable feedback using our proprietary eval metrics.
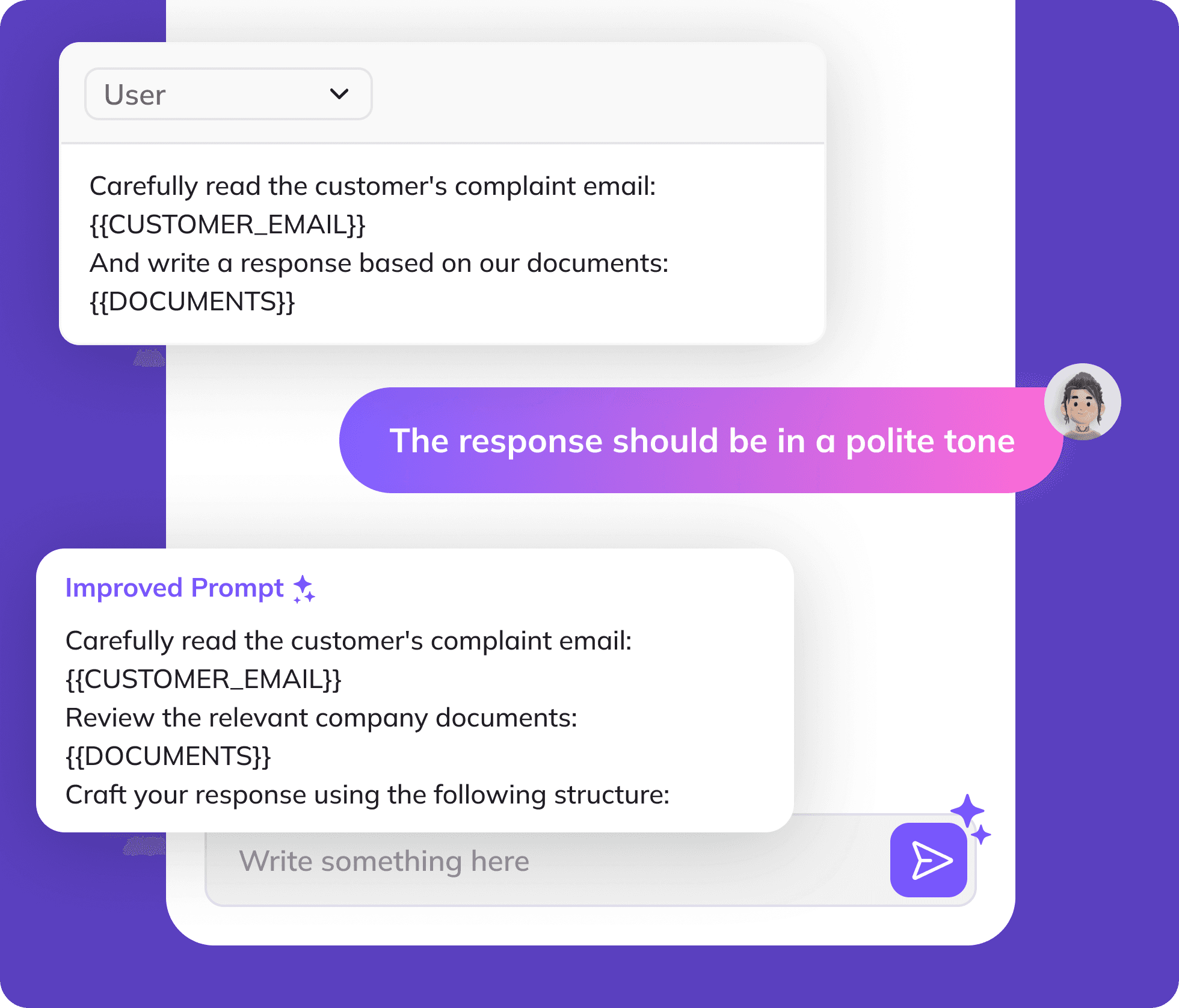
Enhance your LLM application's performance by incorporating feedback from evaluations or custom input, and let system automatically refine your prompt based.
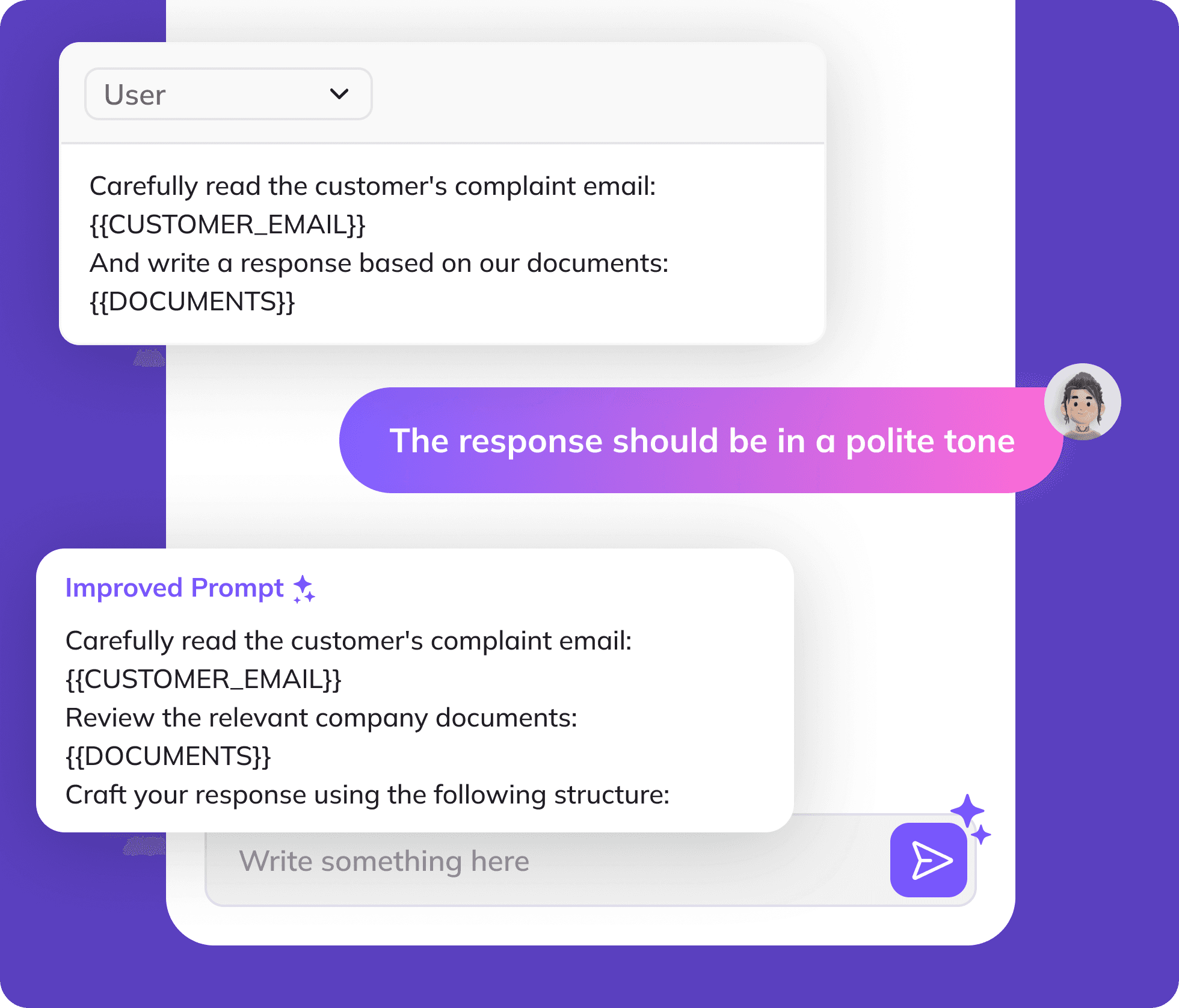
Enhance your LLM application's performance by incorporating feedback from evaluations or custom input, and let system automatically refine your prompt based.
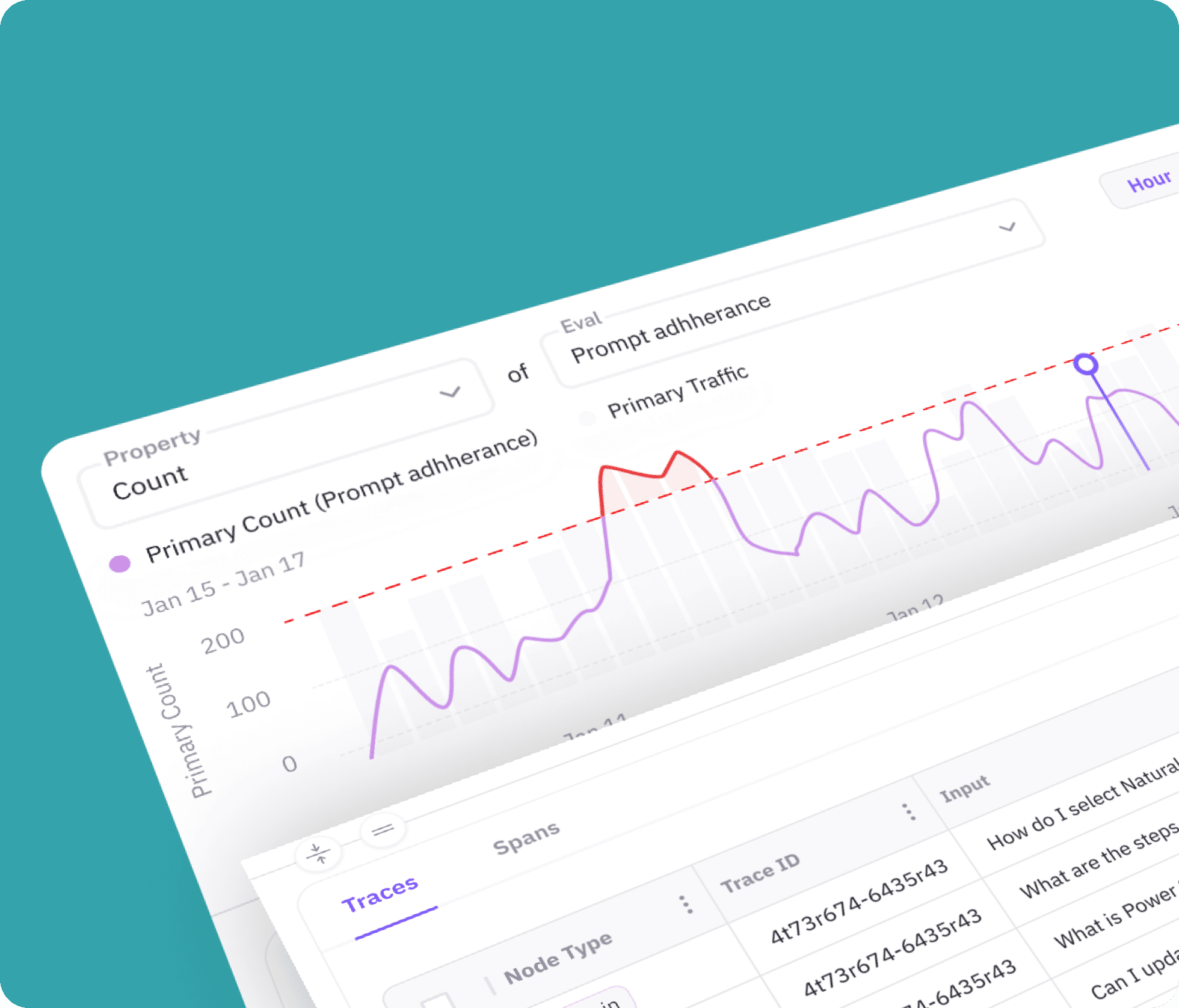
Track applications in production with real-time insights, diagnose issues, and improve robustness, while gaining priority access to Future AGI's safety metrics to block unsafe content with minimal latency.
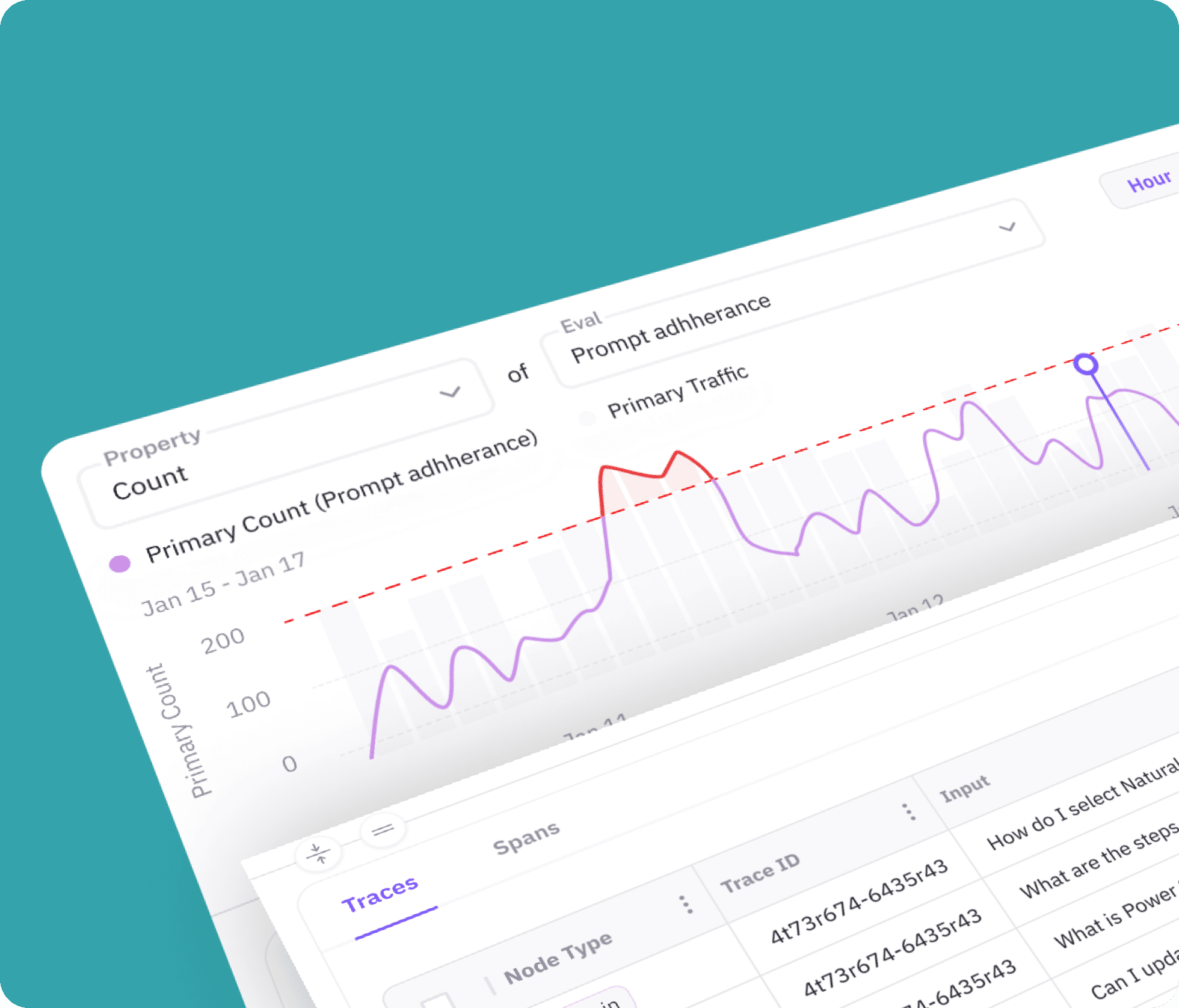
Track applications in production with real-time insights, diagnose issues, and improve robustness, while gaining priority access to Future AGI's safety metrics to block unsafe content with minimal latency.
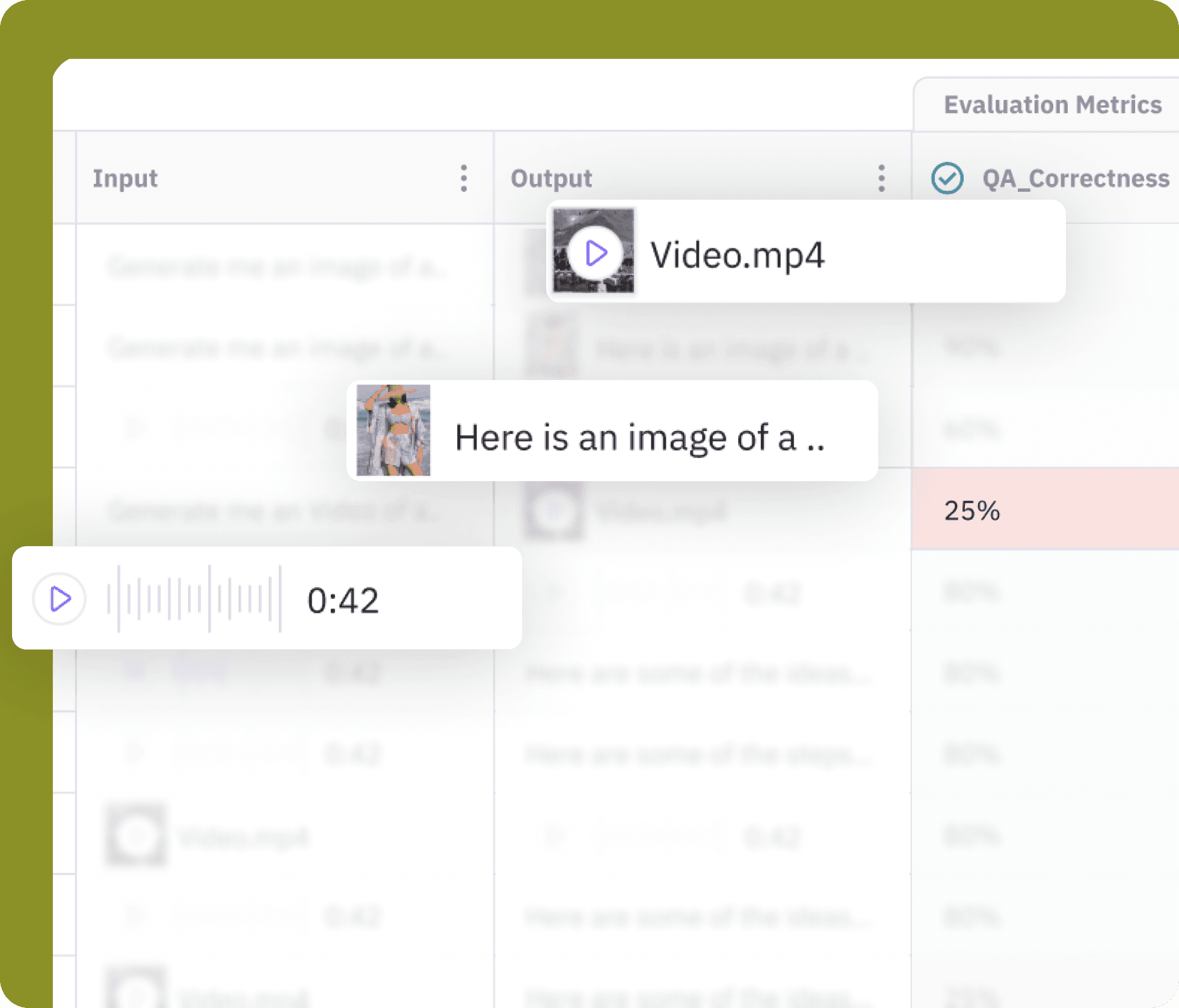
Evaluate your AI across different modalities- text, image, audio, and video. Pinpoint errors and automatically get the feedback to improve it.
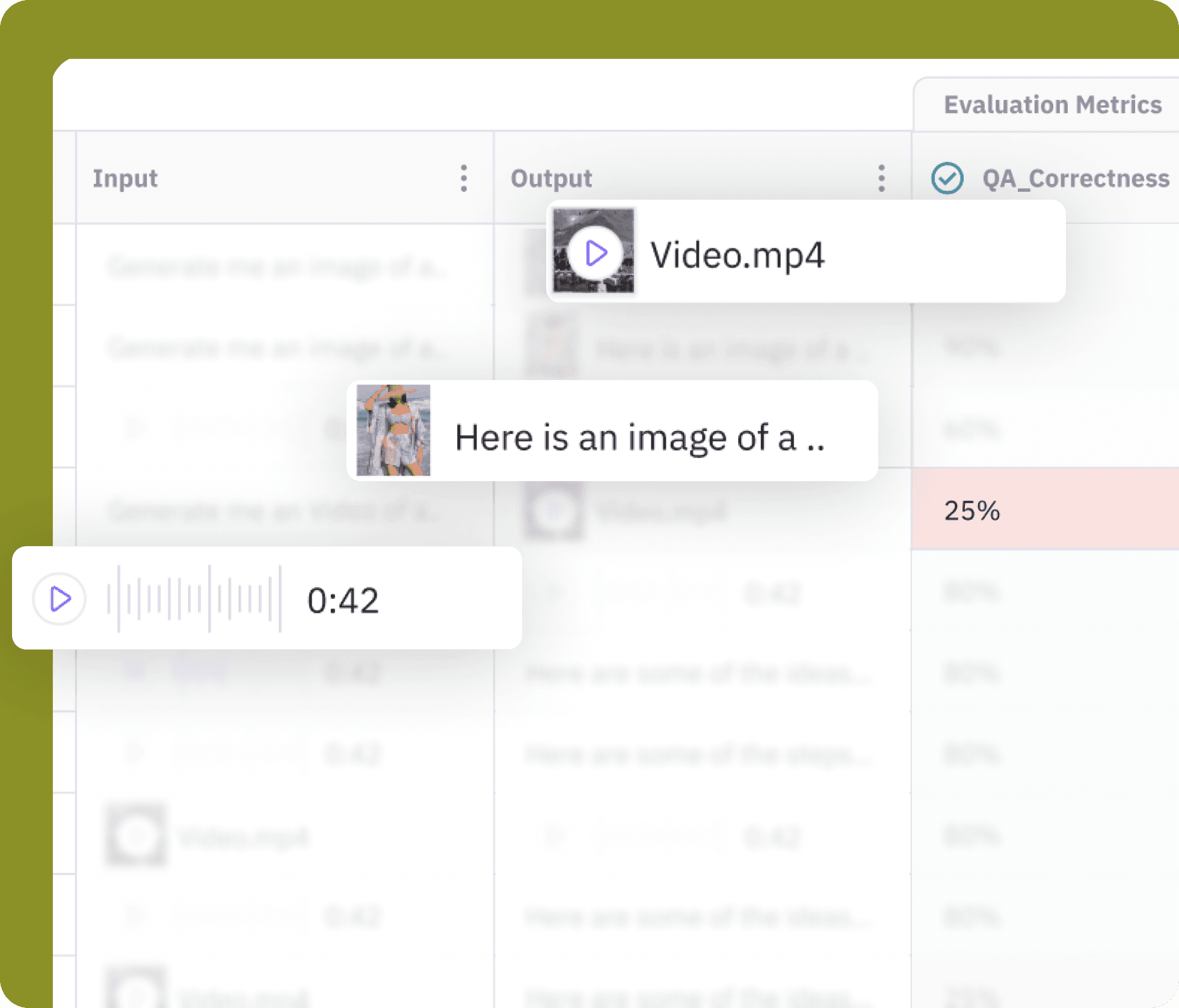
Evaluate your AI across different modalities- text, image, audio, and video. Pinpoint errors and automatically get the feedback to improve it.
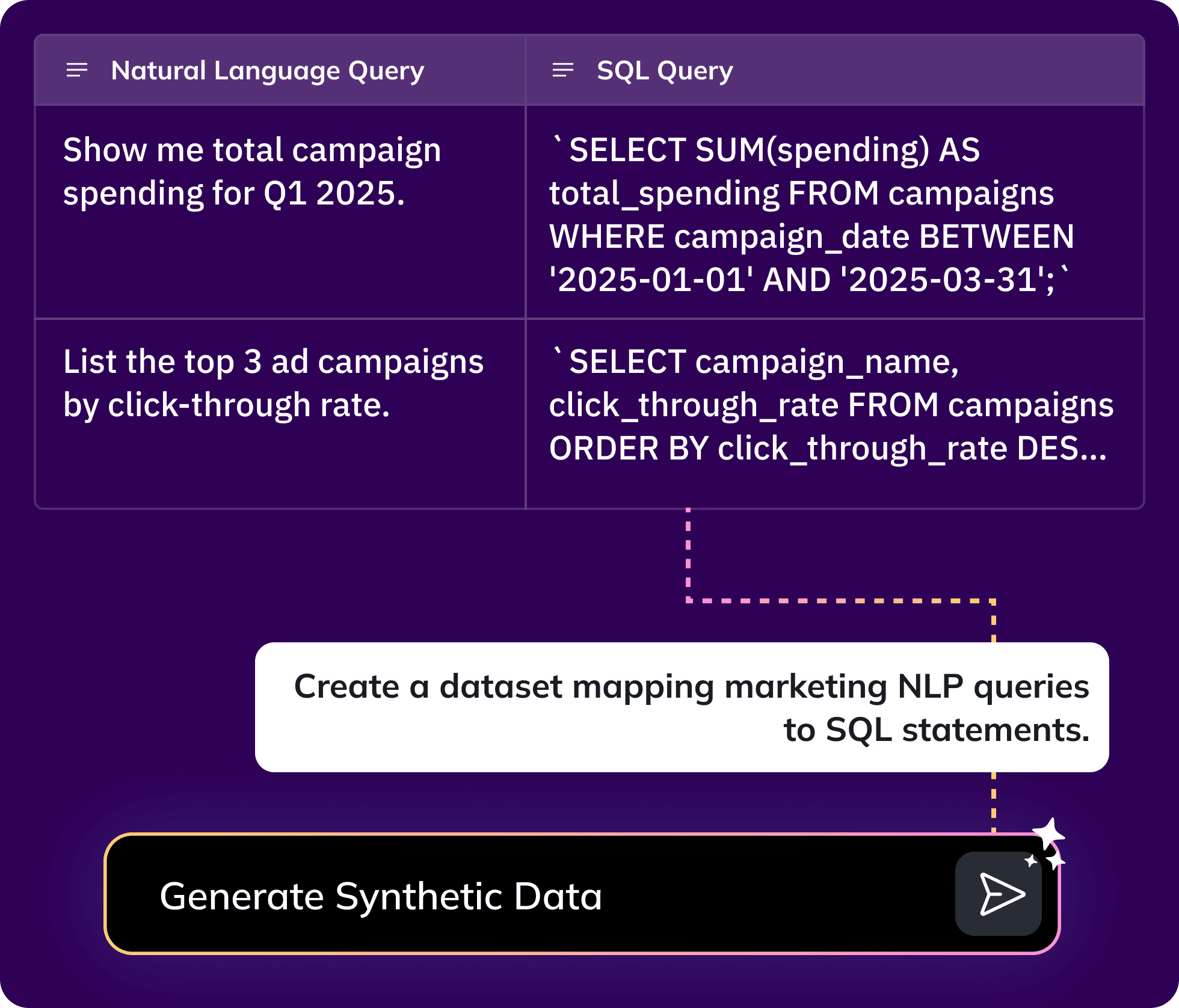
Generate and manage diverse synthetic datasets to effectively train and test AI models, including edge cases.
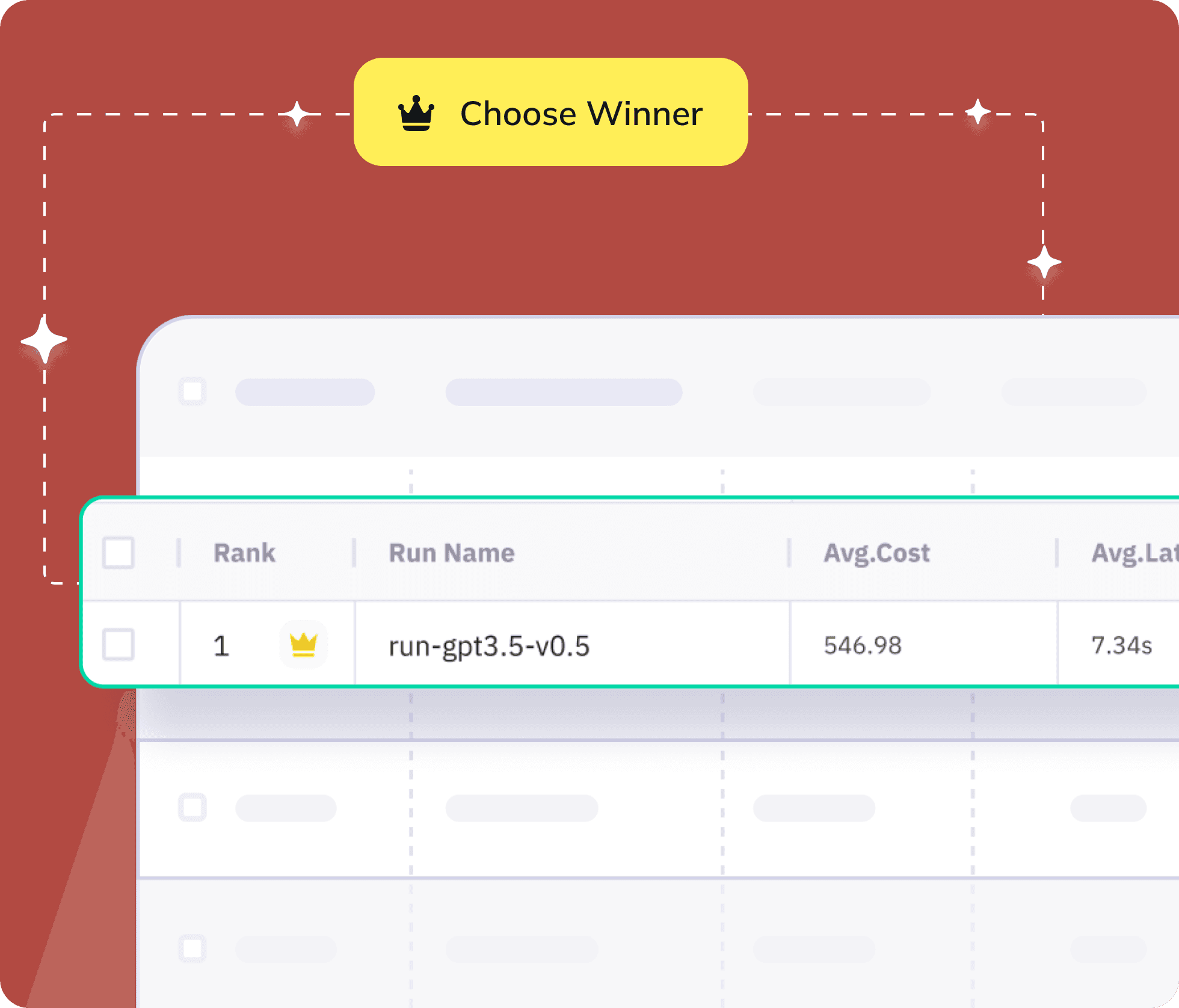
Test, compare and analyse multiple agentic workflow configurations to identify the ‘Winner’ based on built-in or custom evaluation metrics- literally no code!
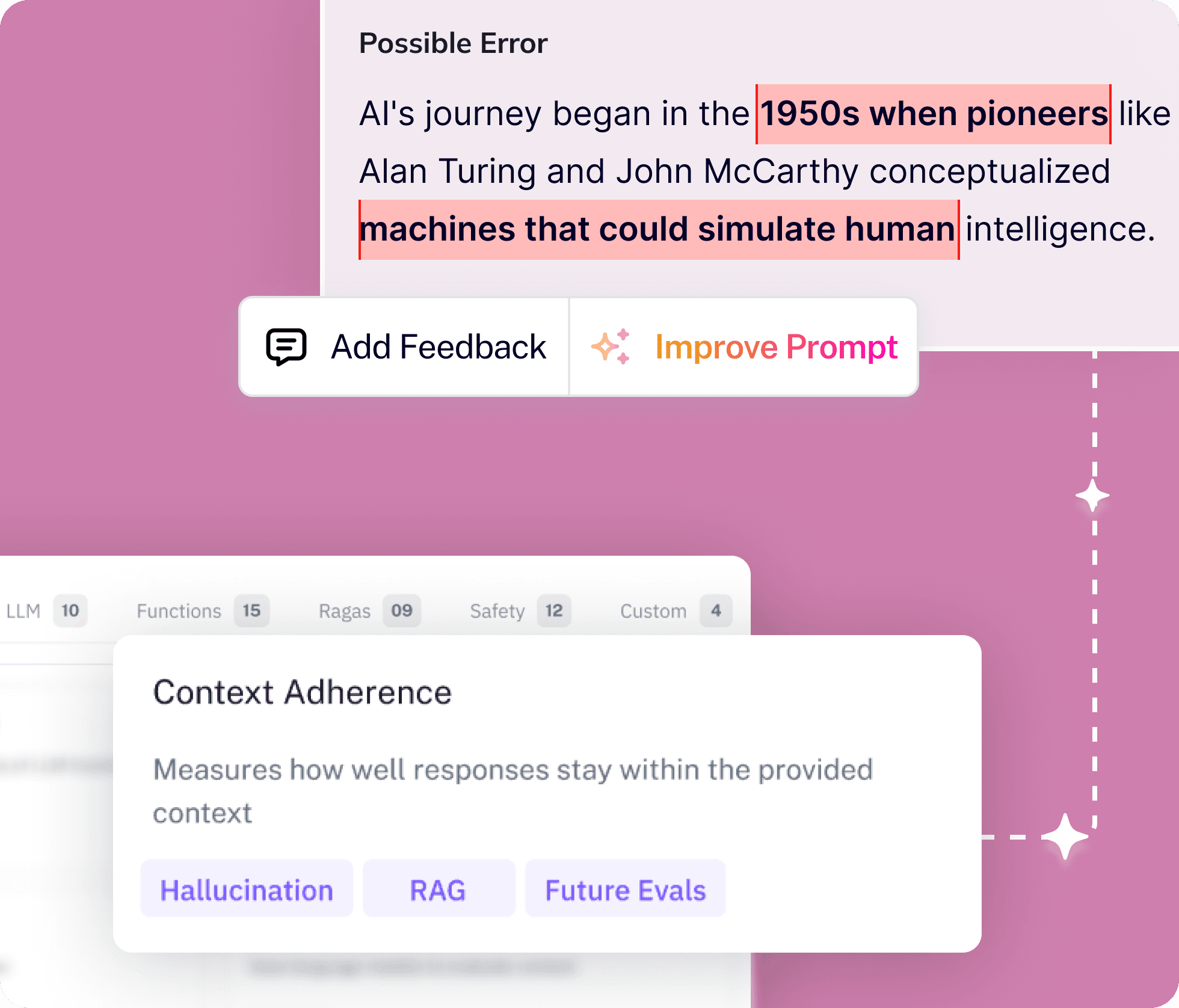
Assess and measure agent performance, pin-point root cause and close loop with actionable feedback using our proprietary eval metrics.
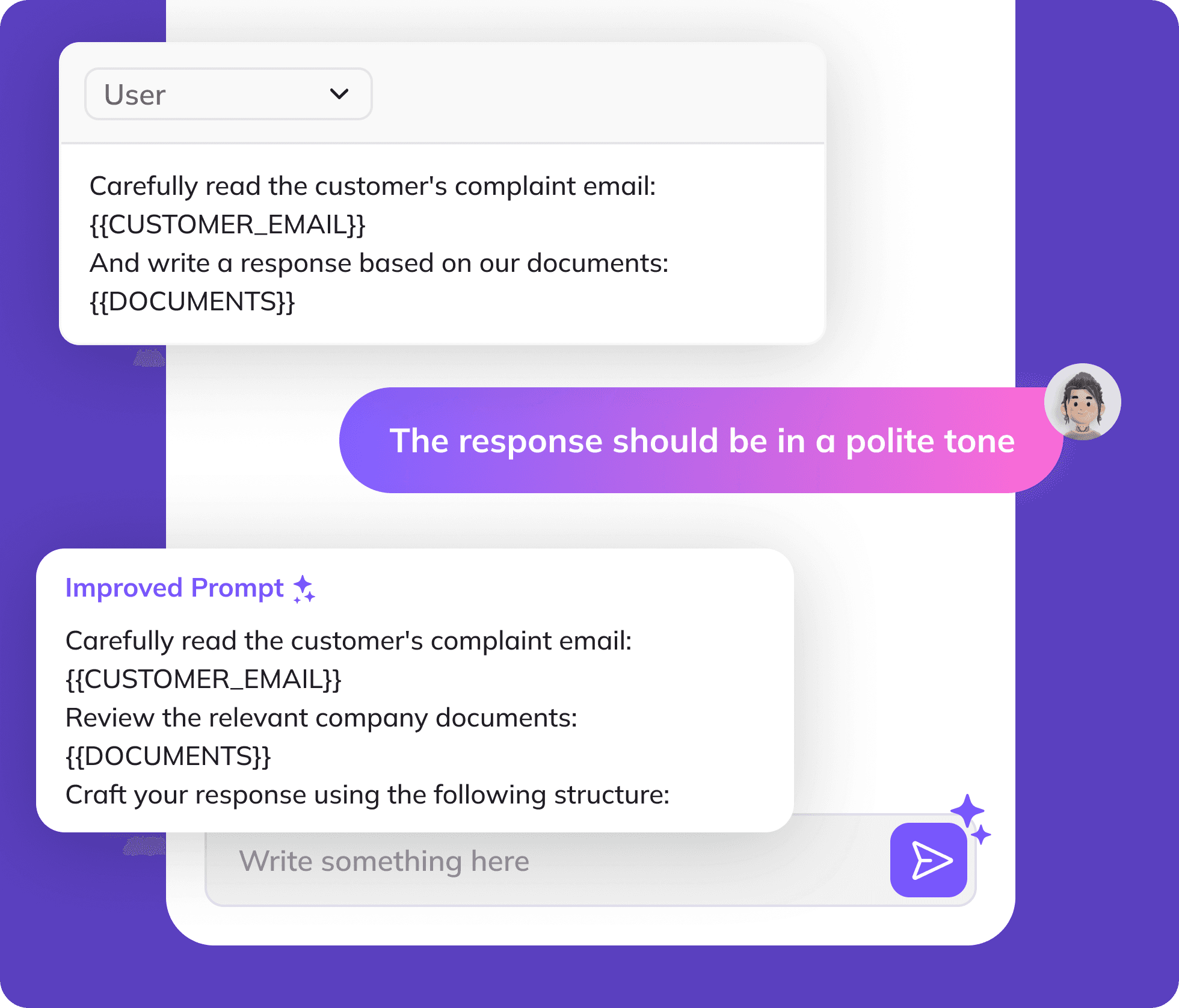
Enhance your LLM application's performance by incorporating feedback from evaluations or custom input, and let system automatically refine your prompt based.
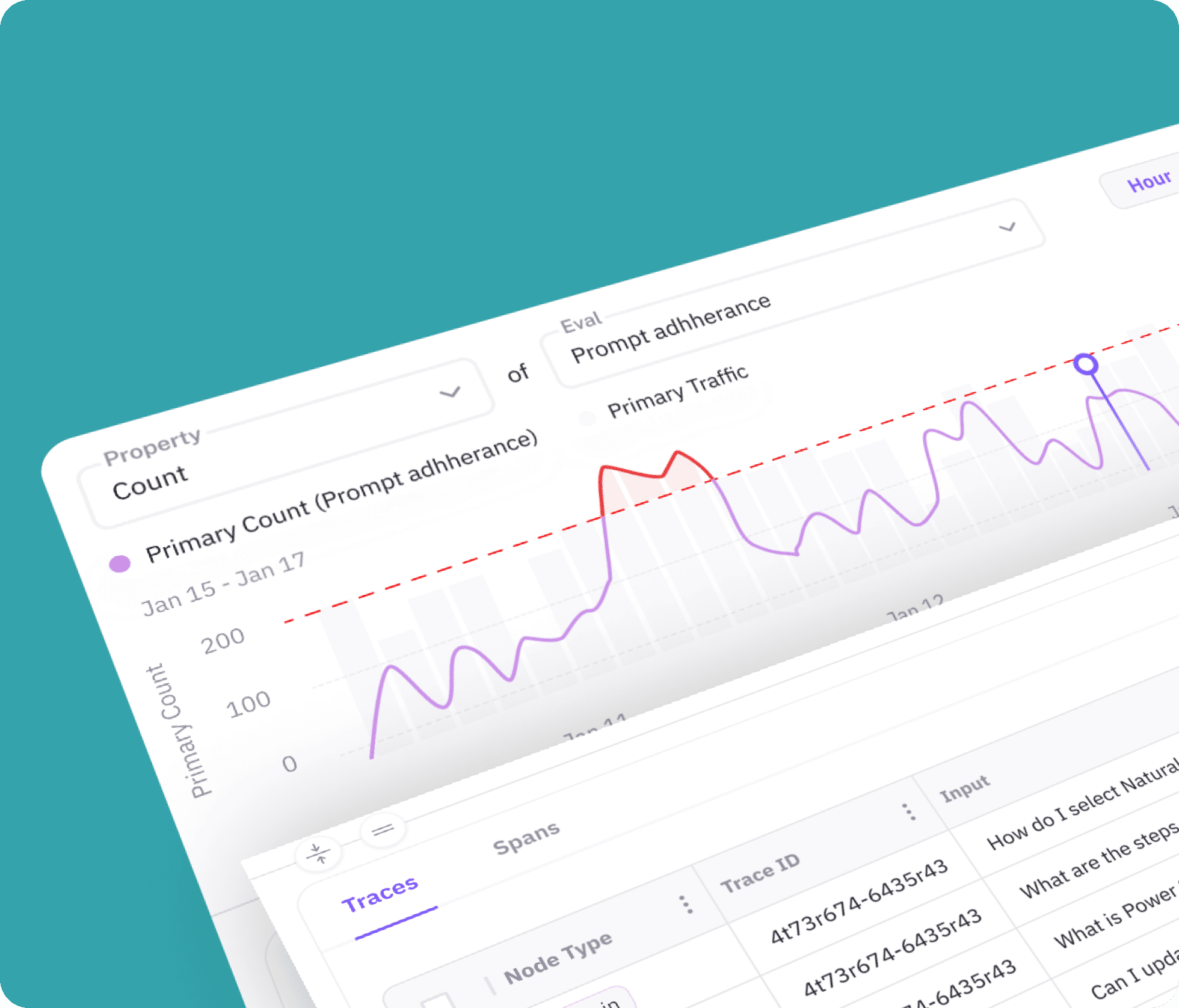
Track applications in production with real-time insights, diagnose issues, and improve robustness, while gaining priority access to Future AGI's safety metrics to block unsafe content with minimal latency.
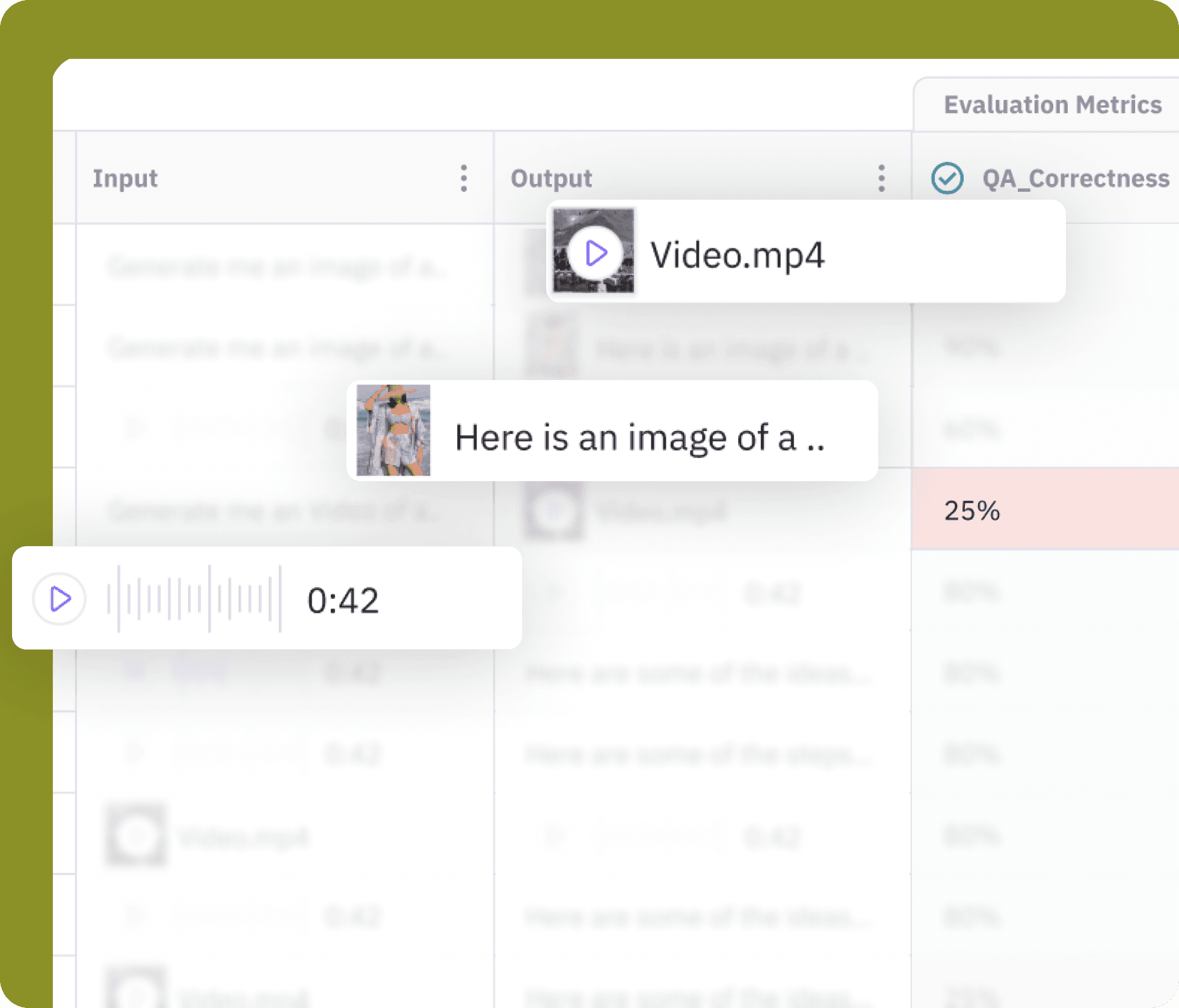
Evaluate your AI across different modalities- text, image, audio, and video. Pinpoint errors and automatically get the feedback to improve it.
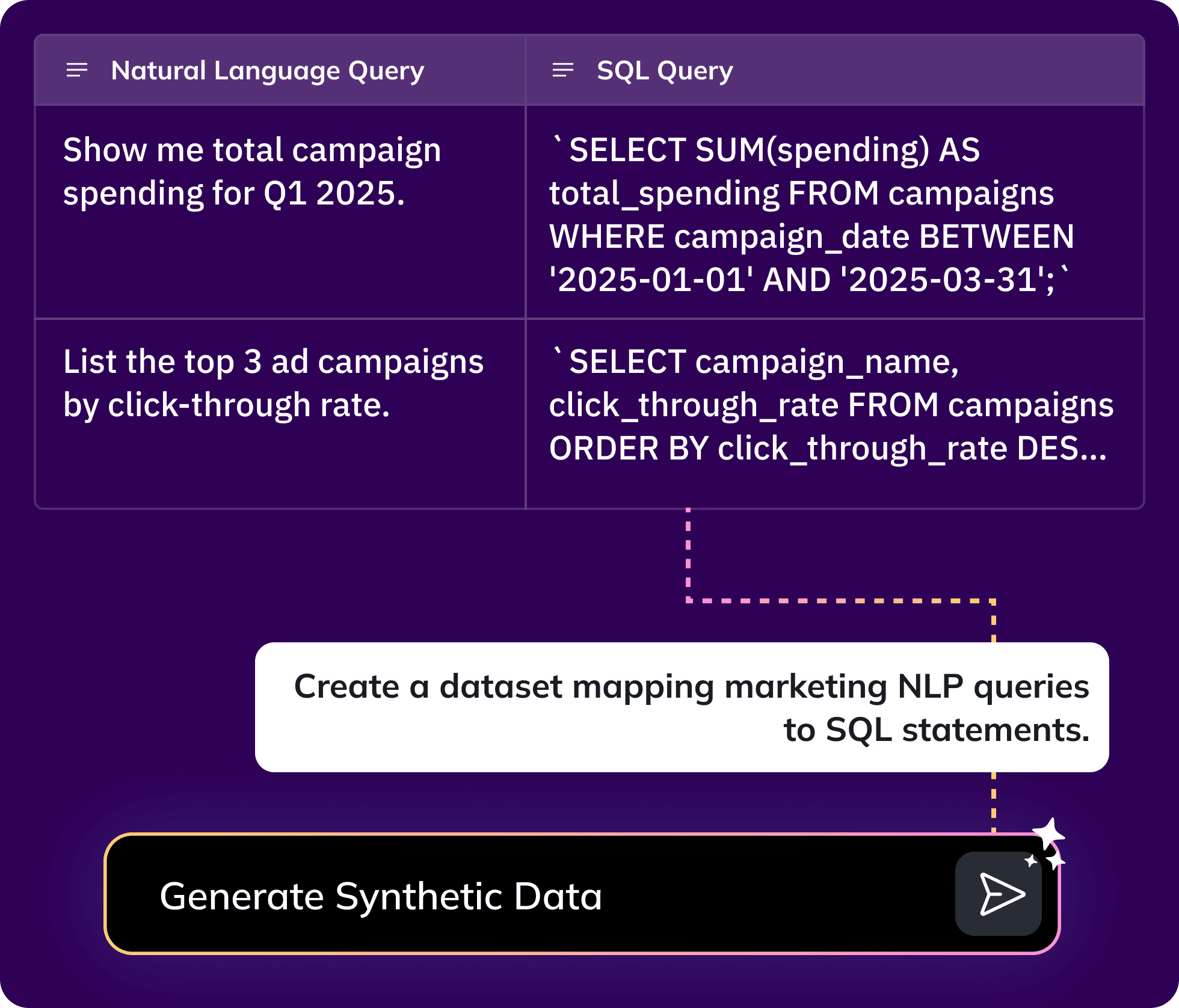
Generate and manage diverse synthetic datasets to effectively train and test AI models, including edge cases.
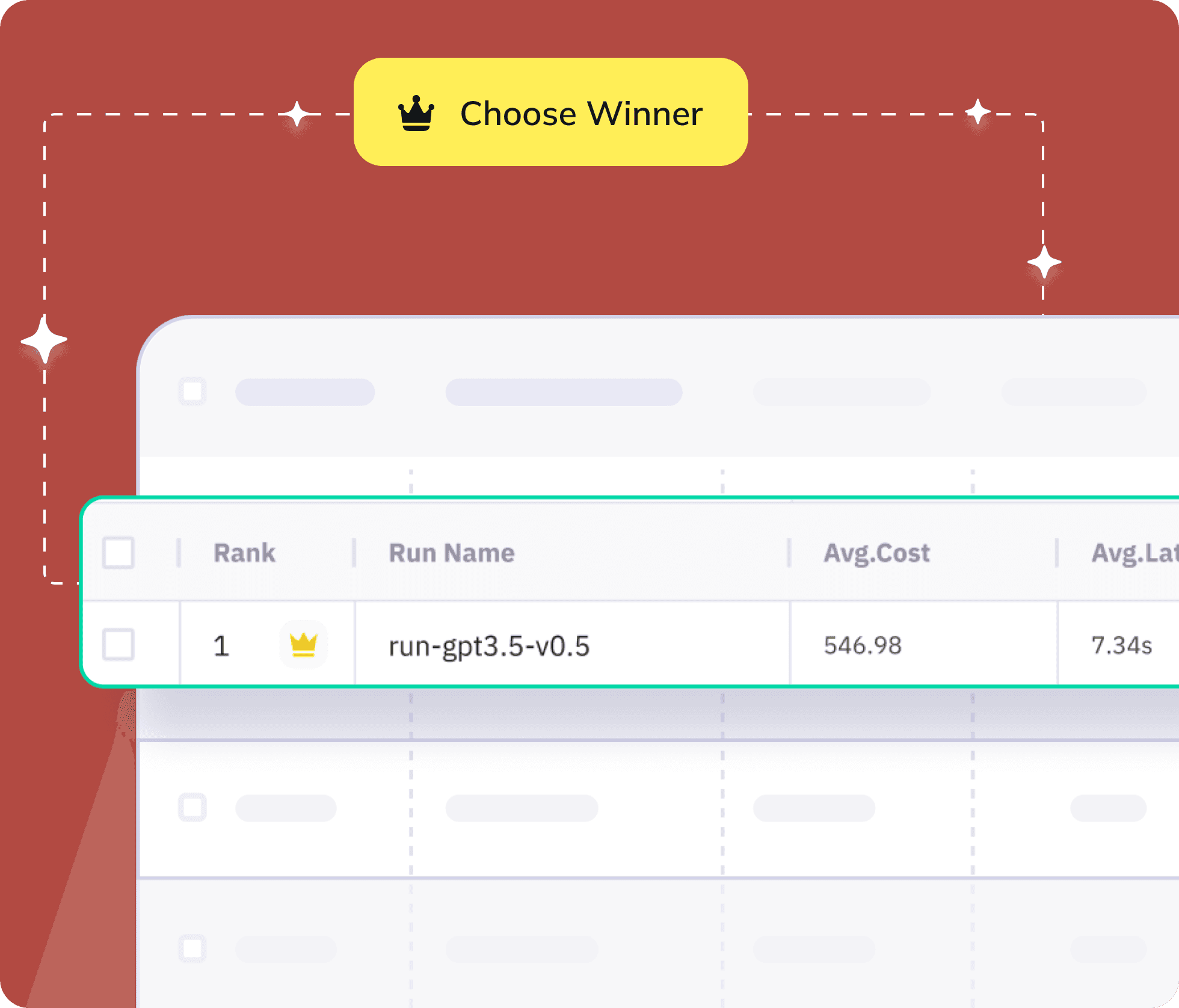
Test, compare and analyse multiple agentic workflow configurations to identify the ‘Winner’ based on built-in or custom evaluation metrics- literally no code!
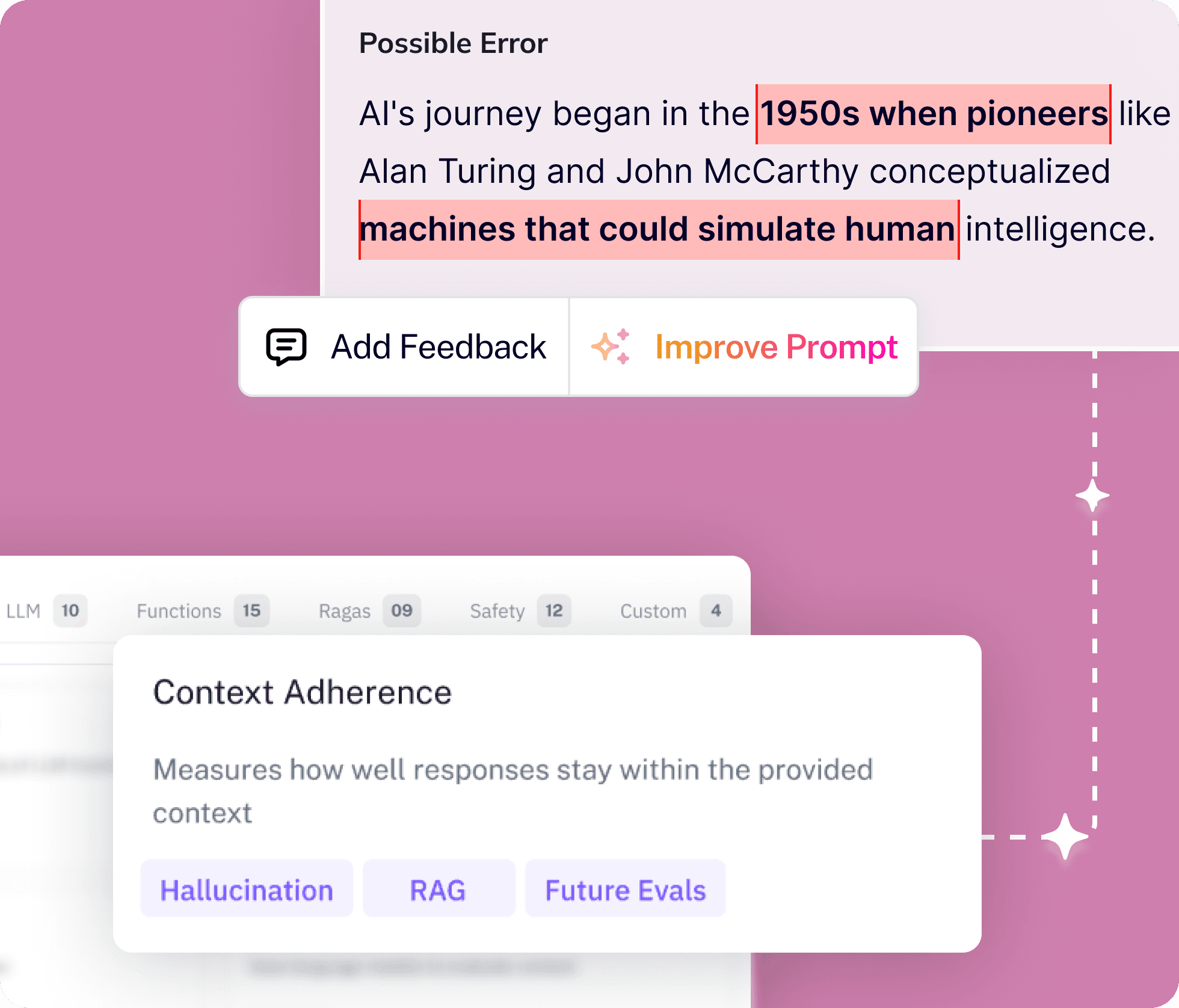
Assess and measure agent performance, pin-point root cause and close the loop with actionable feedback using our proprietary eval metrics.
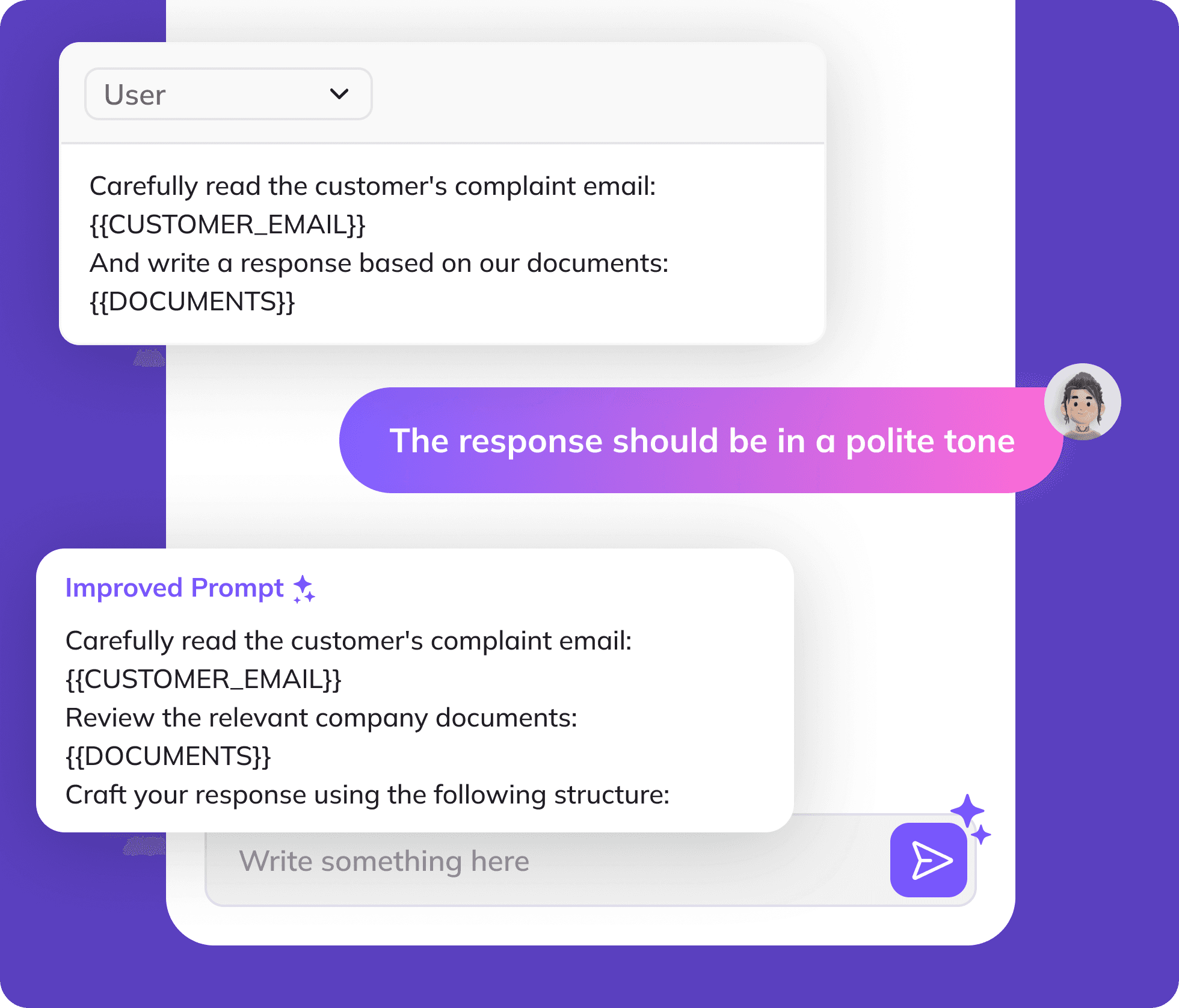
Enhance your LLM application's performance by incorporating feedback from evaluations or custom input, and let our system automatically refine your prompt.
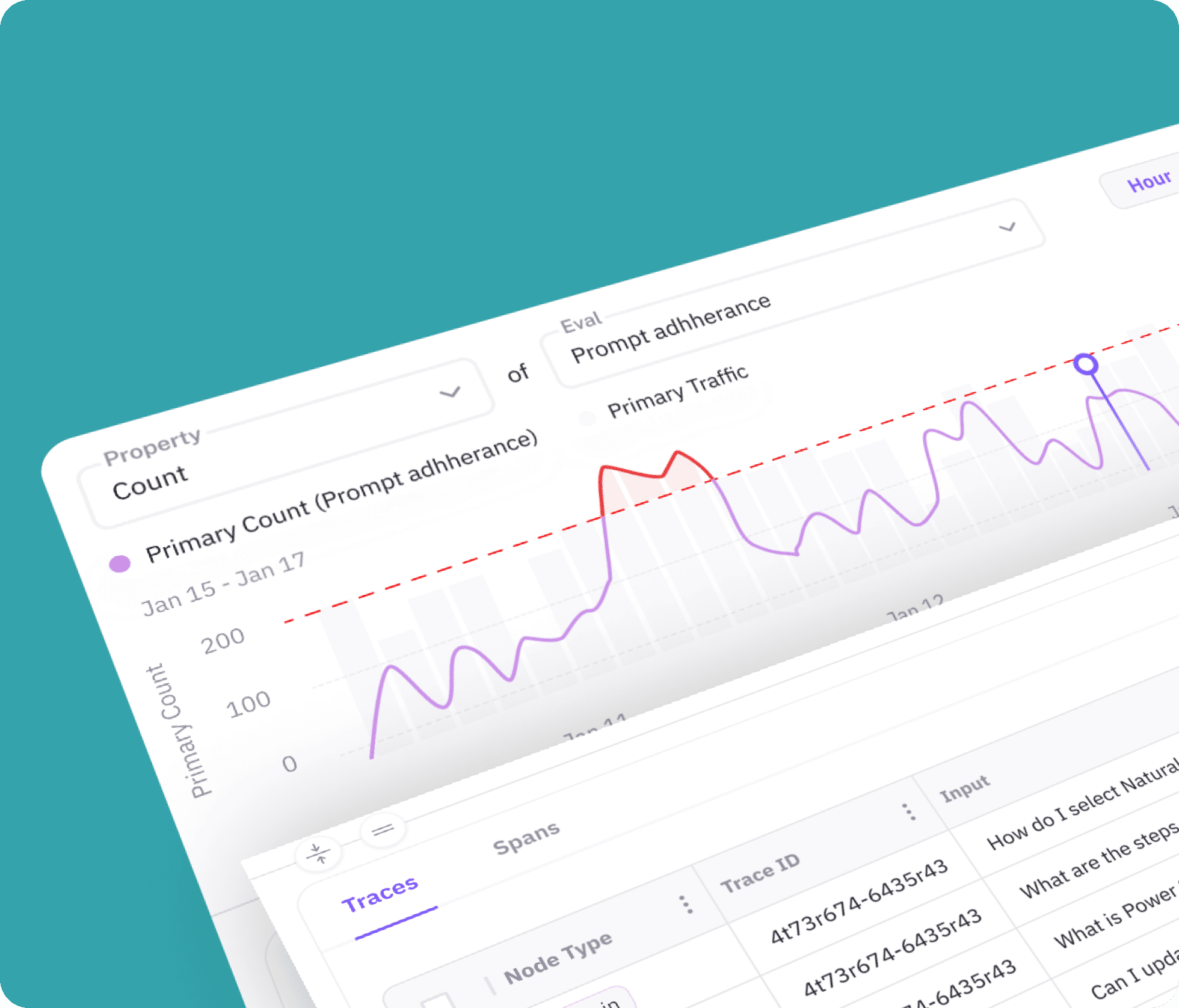
Track applications in production with real-time insights, diagnose issues, and improve robustness, while gaining priority access to Future AGI's safety metrics to block unsafe content with minimal latency.
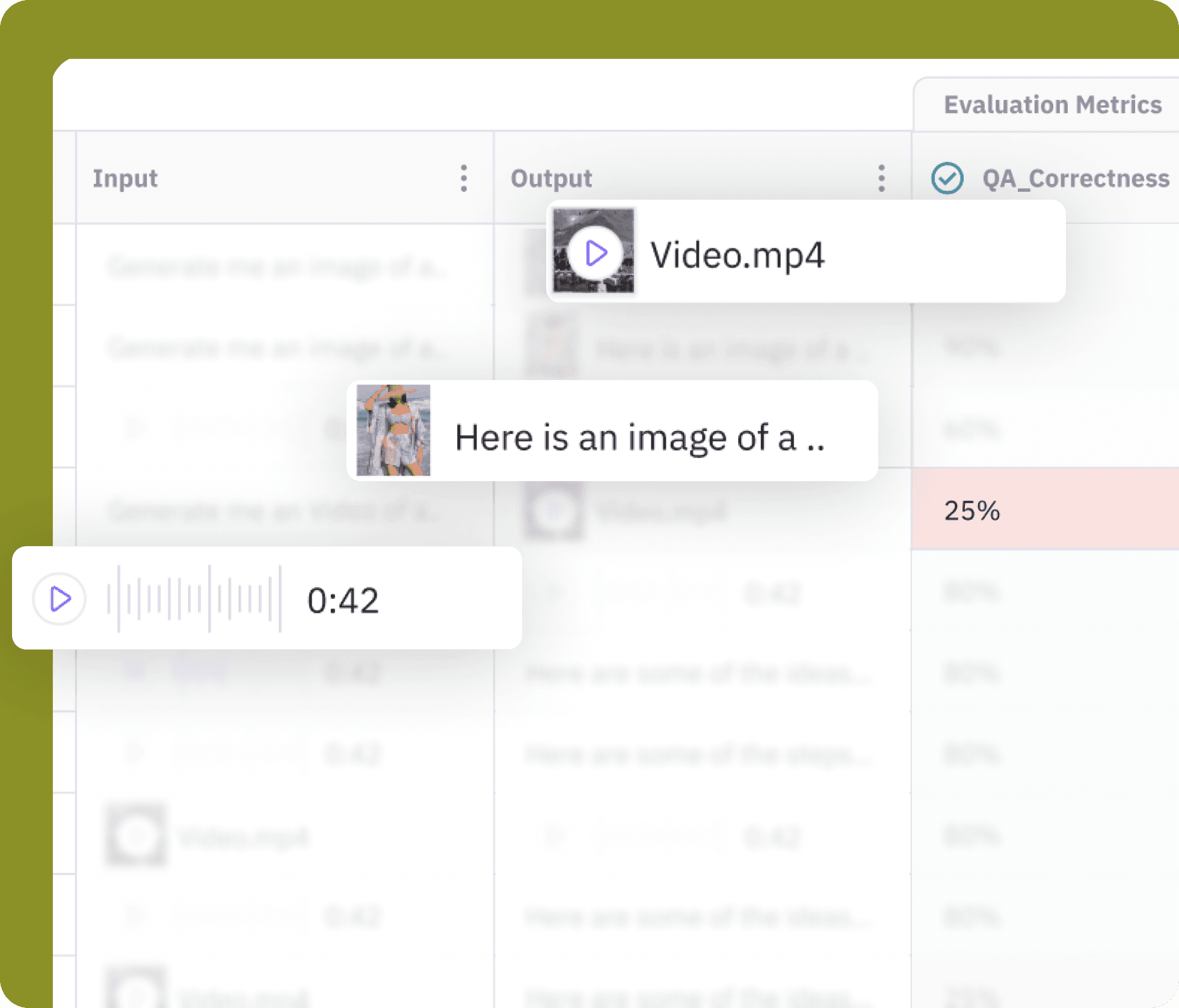
Evaluate your AI across different modalities- text, image, audio, and video. Pinpoint errors and automatically get the feedback to improve it.
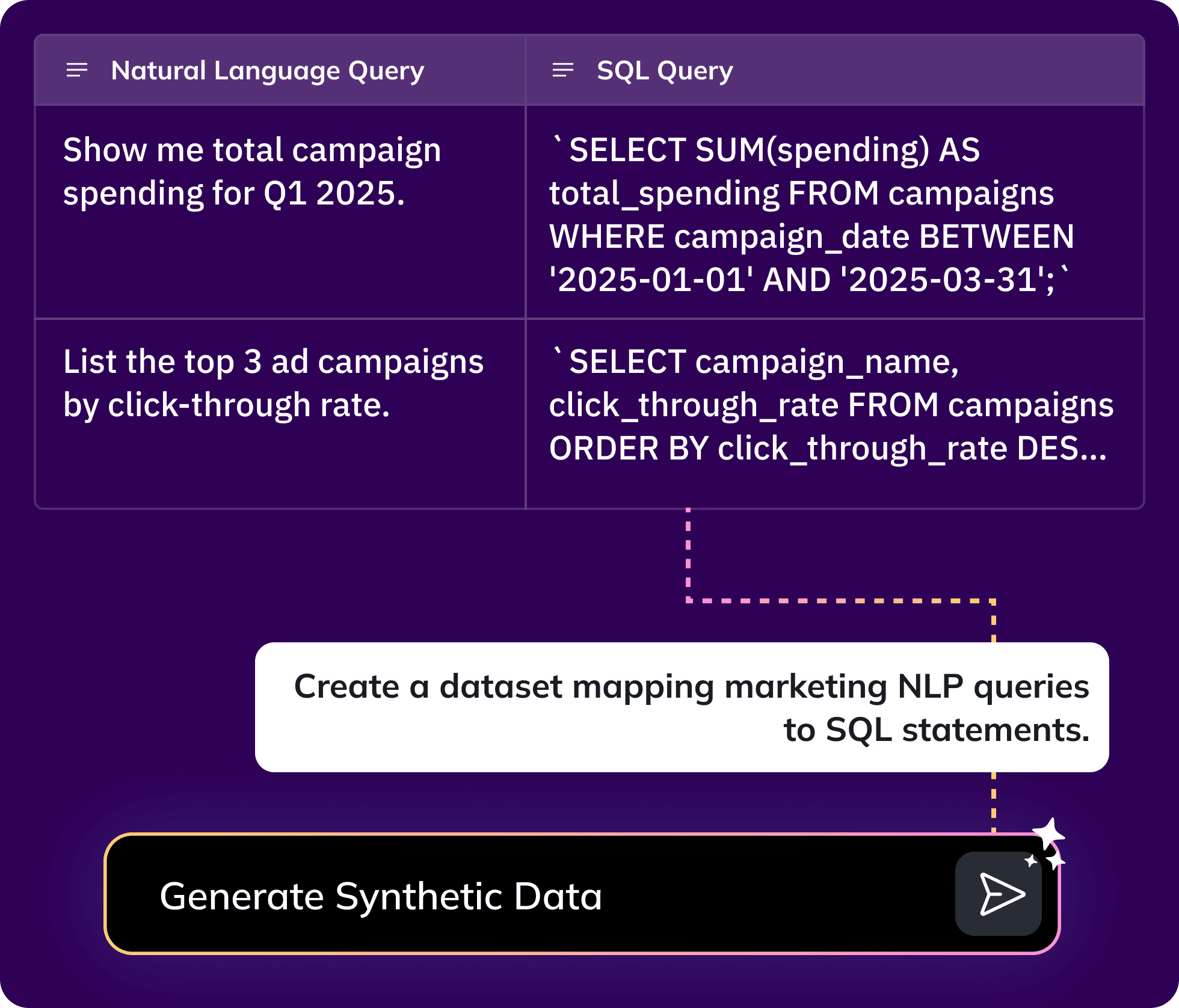
Generate and manage diverse synthetic datasets to effectively train and test AI models, including edge cases.
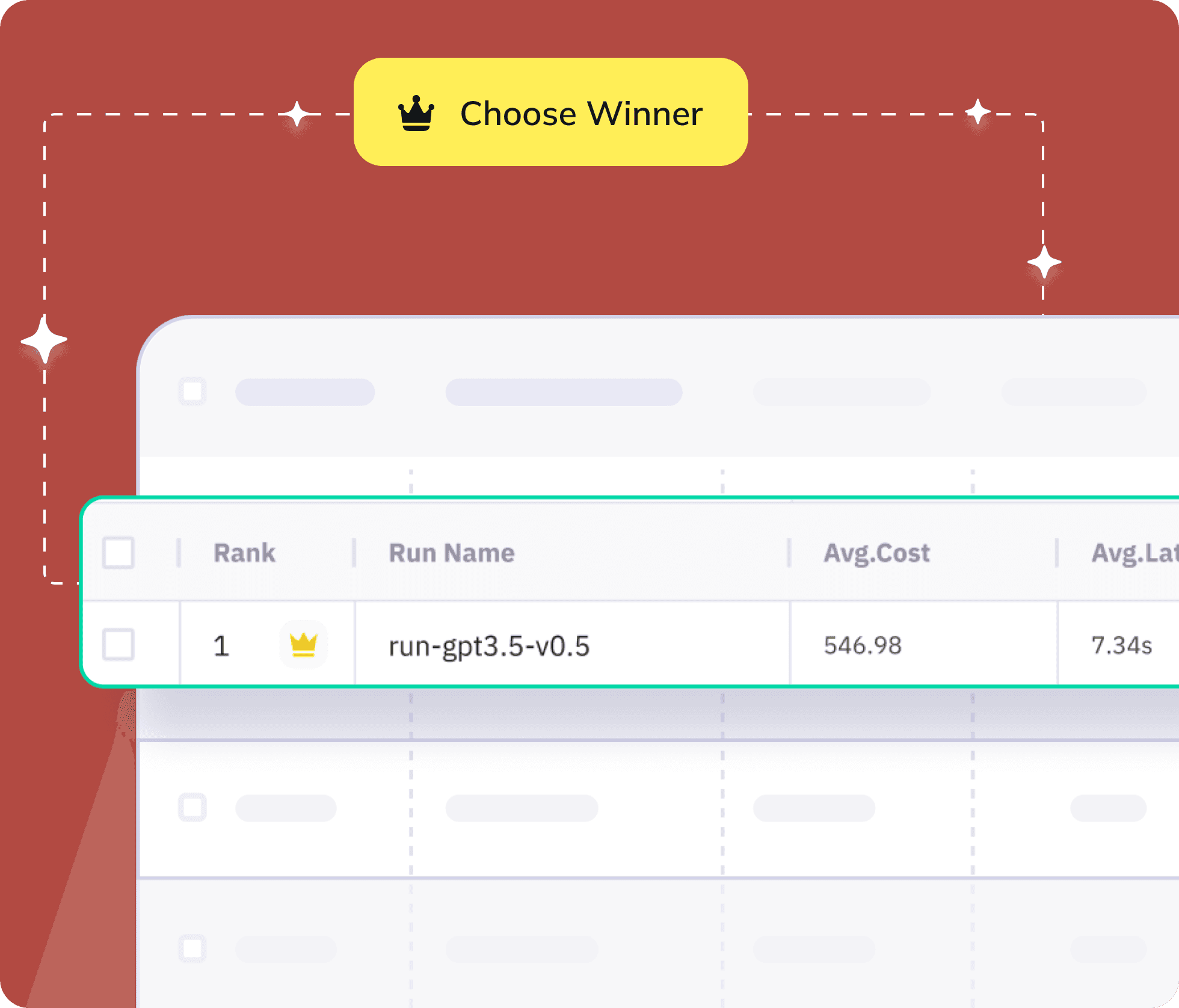
Test, compare and analyse multiple agentic workflow configurations to identify the ‘Winner’ based on built-in or custom evaluation metrics- literally no code!
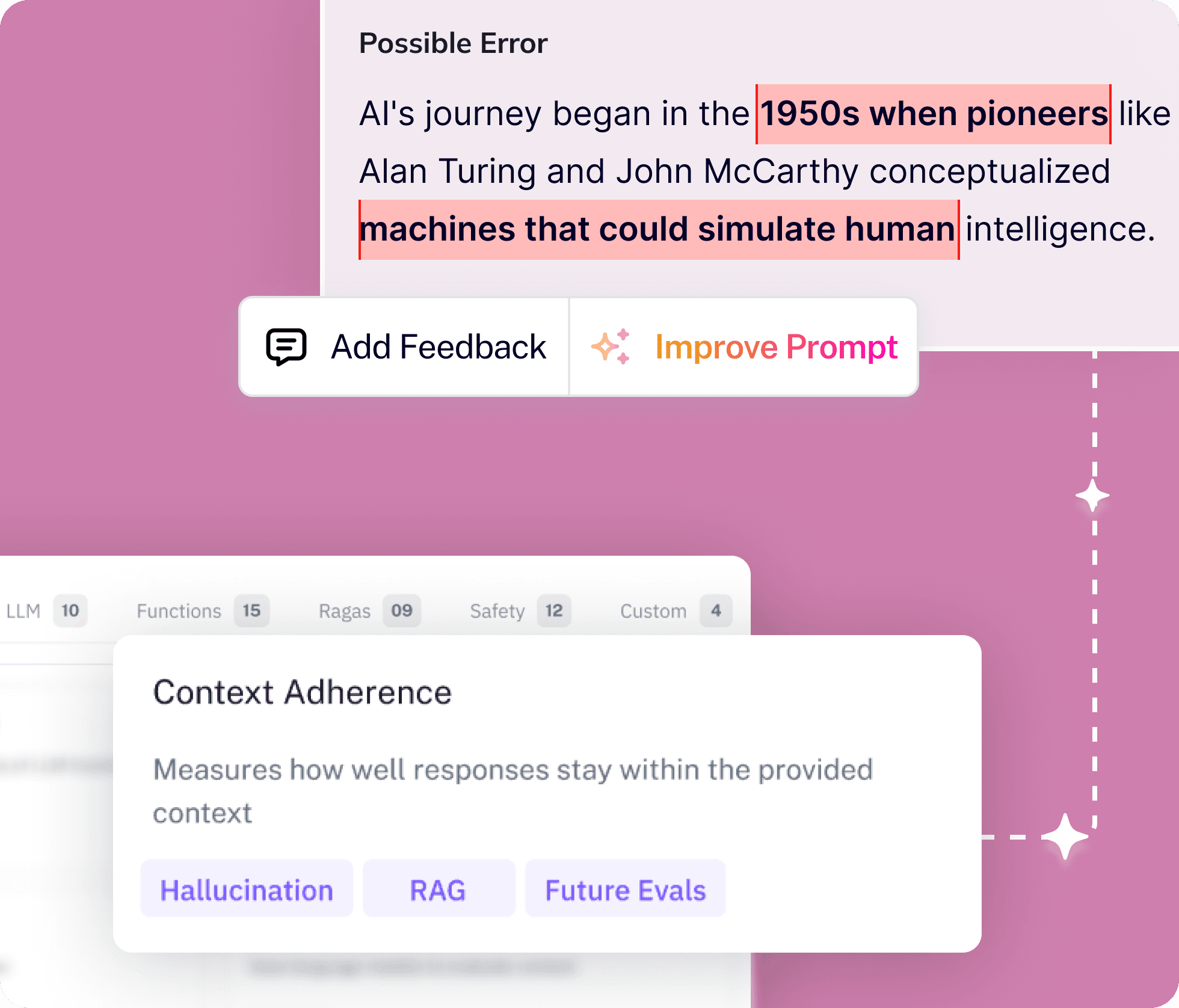
Assess and measure agent performance, pin-point root cause and close loop with actionable feedback using our proprietary eval metrics.
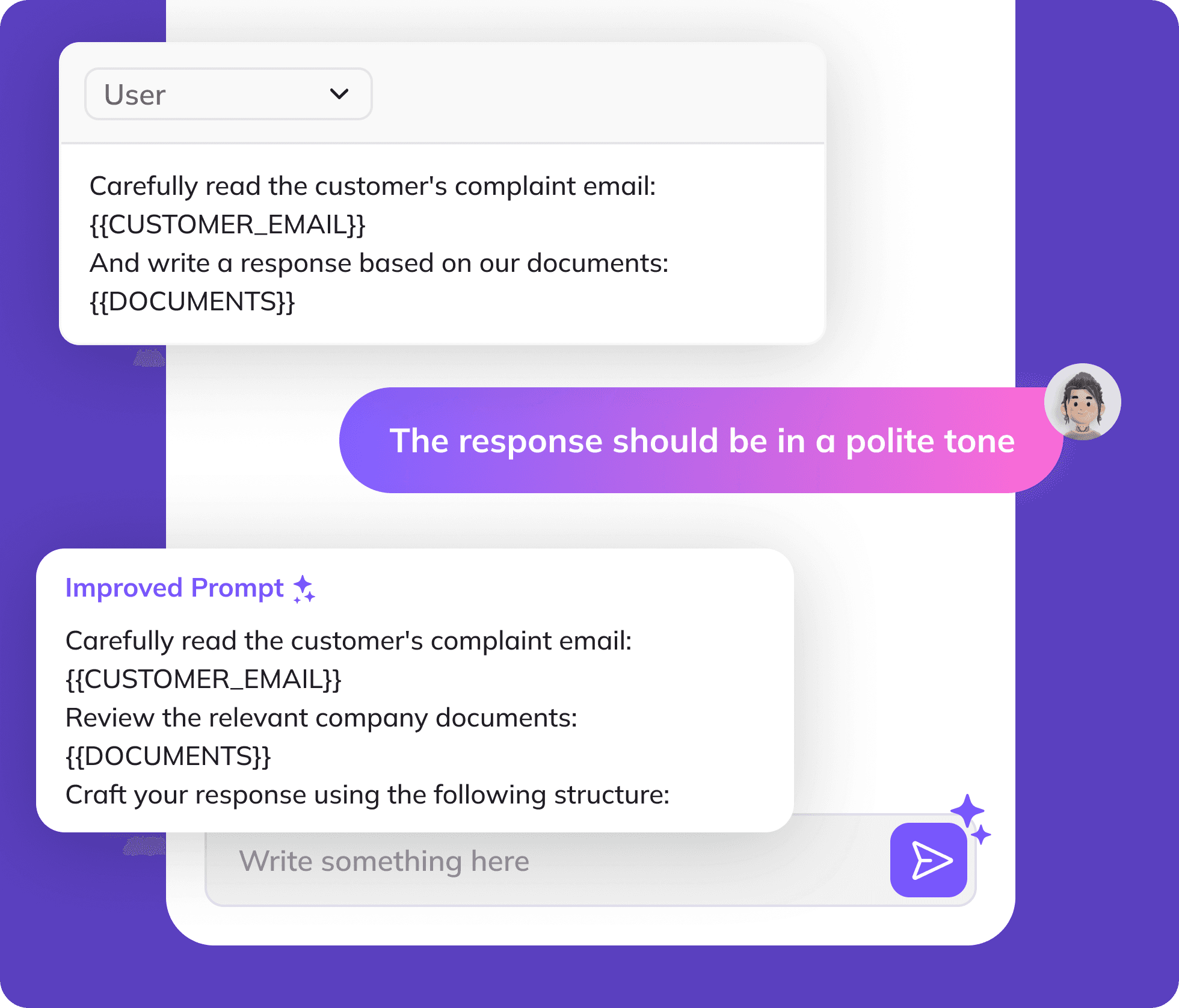
Enhance your LLM application's performance by incorporating feedback from evaluations or custom input, and let system automatically refine your prompt based.
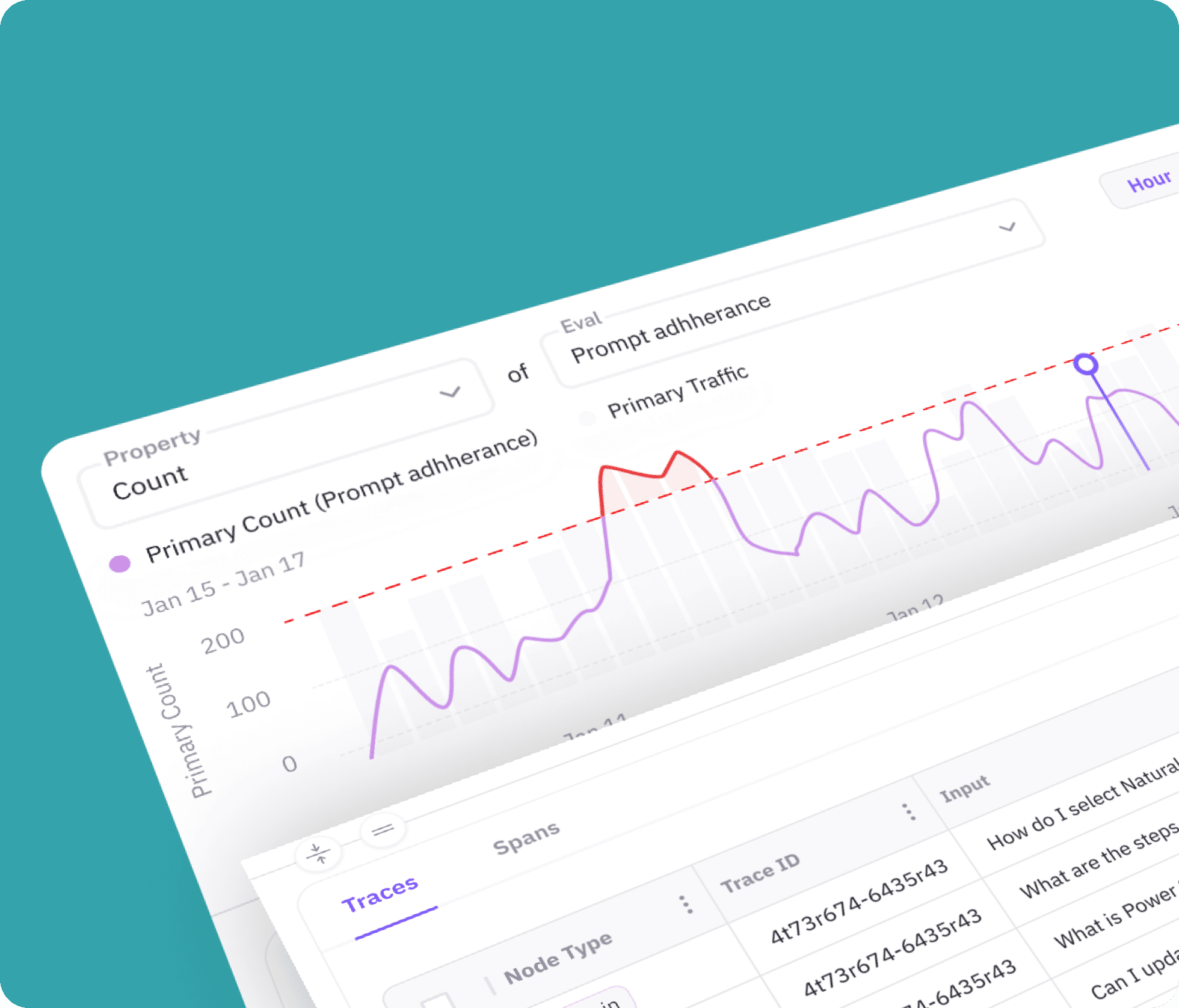
Track applications in production with real-time insights, diagnose issues, and improve robustness, while gaining priority access to Future AGI's safety metrics to block unsafe content with minimal latency.
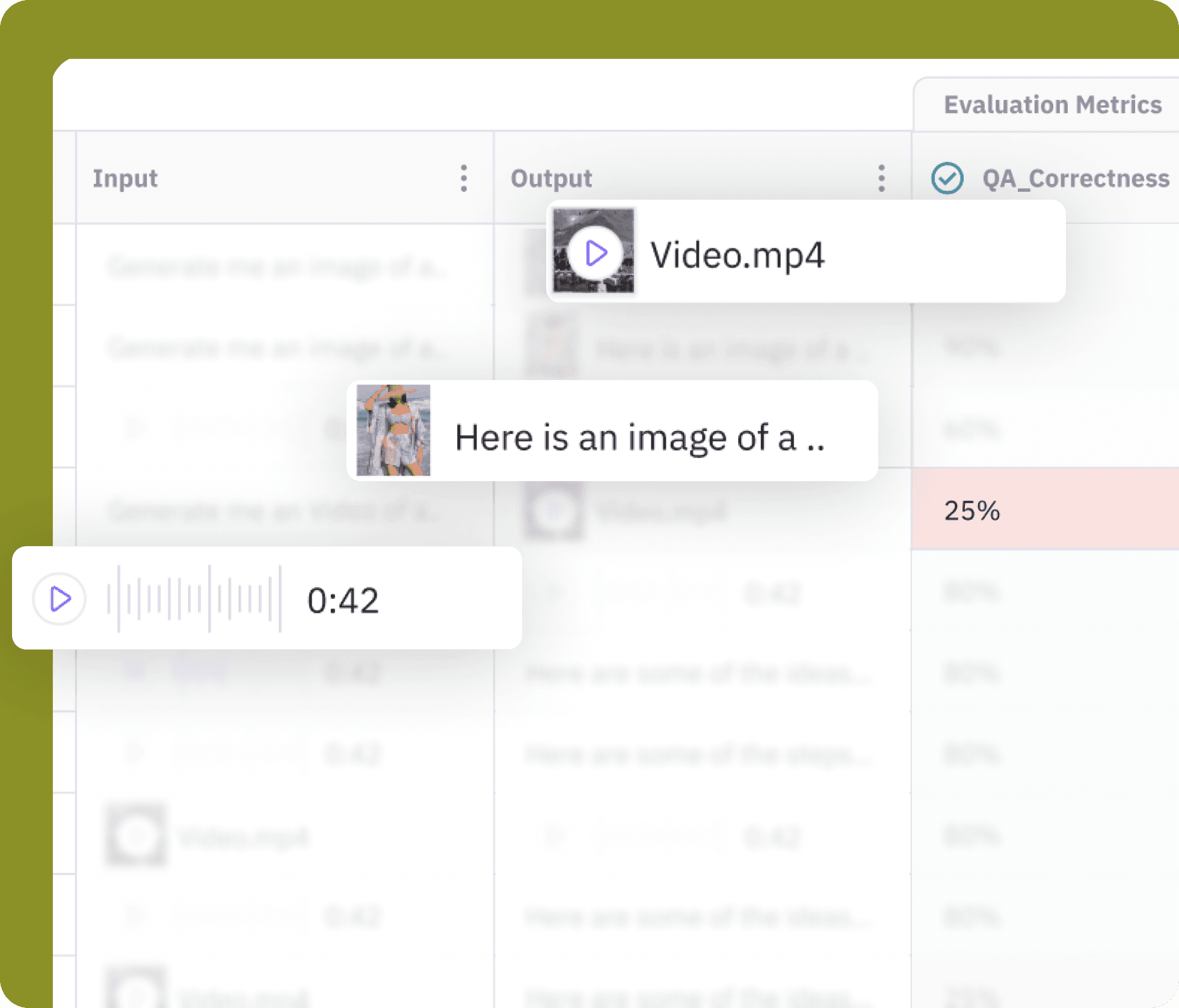
Evaluate your AI across different modalities- text, image, audio, and video. Pinpoint errors and automatically get the feedback to improve it.
Integrate into your Existing Workflow
Integrate into your Existing Workflow
Integrate into your Existing Workflow
Integrate into your Existing Workflow
Future AGI is developer-first and integrates seamlessly with industry-standard tools, so your team can keep their workflow unchanged.
Future AGI is developer-first and integrates seamlessly with industry-standard tools, so your team can keep their workflow unchanged.


# pip install traceAI-openai import os os.environ["OPENAI_API_KEY"] = "your-openai-api-key" os.environ["FI_API_KEY"] = "your-futureagi-api-key" os.environ["FI_SECRET_KEY"] = "your-futureagi-secret-key" from fi_instrumentation import register from fi_instrumentation.fi_types import ProjectType trace_provider = register( project_type=ProjectType.OBSERVE, project_name="openai_project", ) from traceai_openai import OpenAIInstrumentor OpenAIInstrumentor().instrument(tracer_provider=trace_provider) import base64 import httpx from openai import OpenAI client = OpenAI() image_url = "https://upload.wikimedia.org/wikipedia/commons/thumb/d/dd/Gfp-wisconsin-madison-the-nature-boardwalk.jpg/2560px-Gfp-wisconsin-madison-the-nature-boardwalk.jpg" image_media_type = "image/jpeg" image_data = base64.standard_b64encode(httpx.get(image_url).content).decode("utf-8") response = client.chat.completions.create( model="gpt-4o", messages=[ { "role": "user", "content": [ {"type": "text", "text": "What is in this image?"}, { "type": "image_url", "image_url": { "url": "https://upload.wikimedia.org/wikipedia/commons/thumb/d/dd/Gfp-wisconsin-madison-the-nature-boardwalk.jpg/2560px-Gfp-wisconsin-madison-the-nature-boardwalk.jpg", }, }, ], }, ], ) print(response.choices[0].message.content)


# pip install traceAI-openai import os os.environ["OPENAI_API_KEY"] = "your-openai-api-key" os.environ["FI_API_KEY"] = "your-futureagi-api-key" os.environ["FI_SECRET_KEY"] = "your-futureagi-secret-key" from fi_instrumentation import register from fi_instrumentation.fi_types import ProjectType trace_provider = register( project_type=ProjectType.OBSERVE, project_name="openai_project", ) from traceai_openai import OpenAIInstrumentor OpenAIInstrumentor().instrument(tracer_provider=trace_provider) import base64 import httpx from openai import OpenAI client = OpenAI() image_url = "https://upload.wikimedia.org/wikipedia/commons/thumb/d/dd/Gfp-wisconsin-madison-the-nature-boardwalk.jpg/2560px-Gfp-wisconsin-madison-the-nature-boardwalk.jpg" image_media_type = "image/jpeg" image_data = base64.standard_b64encode(httpx.get(image_url).content).decode("utf-8") response = client.chat.completions.create( model="gpt-4o", messages=[ { "role": "user", "content": [ {"type": "text", "text": "What is in this image?"}, { "type": "image_url", "image_url": { "url": "https://upload.wikimedia.org/wikipedia/commons/thumb/d/dd/Gfp-wisconsin-madison-the-nature-boardwalk.jpg/2560px-Gfp-wisconsin-madison-the-nature-boardwalk.jpg", }, }, ], }, ], ) print(response.choices[0].message.content)


# pip install traceAI-openai import os os.environ["OPENAI_API_KEY"] = "your-openai-api-key" os.environ["FI_API_KEY"] = "your-futureagi-api-key" os.environ["FI_SECRET_KEY"] = "your-futureagi-secret-key" from fi_instrumentation import register from fi_instrumentation.fi_types import ProjectType trace_provider = register( project_type=ProjectType.OBSERVE, project_name="openai_project", ) from traceai_openai import OpenAIInstrumentor OpenAIInstrumentor().instrument(tracer_provider=trace_provider) import base64 import httpx from openai import OpenAI client = OpenAI() image_url = "https://upload.wikimedia.org/wikipedia/commons/thumb/d/dd/Gfp-wisconsin-madison-the-nature-boardwalk.jpg/2560px-Gfp-wisconsin-madison-the-nature-boardwalk.jpg" image_media_type = "image/jpeg" image_data = base64.standard_b64encode(httpx.get(image_url).content).decode("utf-8") response = client.chat.completions.create( model="gpt-4o", messages=[ { "role": "user", "content": [ {"type": "text", "text": "What is in this image?"}, { "type": "image_url", "image_url": { "url": "https://upload.wikimedia.org/wikipedia/commons/thumb/d/dd/Gfp-wisconsin-madison-the-nature-boardwalk.jpg/2560px-Gfp-wisconsin-madison-the-nature-boardwalk.jpg", }, }, ], }, ], ) print(response.choices[0].message.content)


# pip install traceAI-openai import os os.environ["OPENAI_API_KEY"] = "your-openai-api-key" os.environ["FI_API_KEY"] = "your-futureagi-api-key" os.environ["FI_SECRET_KEY"] = "your-futureagi-secret-key" from fi_instrumentation import register from fi_instrumentation.fi_types import ProjectType trace_provider = register( project_type=ProjectType.OBSERVE, project_name="openai_project", ) from traceai_openai import OpenAIInstrumentor OpenAIInstrumentor().instrument(tracer_provider=trace_provider) import base64 import httpx from openai import OpenAI client = OpenAI() image_url = "https://upload.wikimedia.org/wikipedia/commons/thumb/d/dd/Gfp-wisconsin-madison-the-nature-boardwalk.jpg/2560px-Gfp-wisconsin-madison-the-nature-boardwalk.jpg" image_media_type = "image/jpeg" image_data = base64.standard_b64encode(httpx.get(image_url).content).decode("utf-8") response = client.chat.completions.create( model="gpt-4o", messages=[ { "role": "user", "content": [ {"type": "text", "text": "What is in this image?"}, { "type": "image_url", "image_url": { "url": "https://upload.wikimedia.org/wikipedia/commons/thumb/d/dd/Gfp-wisconsin-madison-the-nature-boardwalk.jpg/2560px-Gfp-wisconsin-madison-the-nature-boardwalk.jpg", }, }, ], }, ], ) print(response.choices[0].message.content)


# pip install traceAI-openai import os os.environ["OPENAI_API_KEY"] = "your-openai-api-key" os.environ["FI_API_KEY"] = "your-futureagi-api-key" os.environ["FI_SECRET_KEY"] = "your-futureagi-secret-key" from fi_instrumentation import register from fi_instrumentation.fi_types import ProjectType trace_provider = register( project_type=ProjectType.OBSERVE, project_name="openai_project", ) from traceai_openai import OpenAIInstrumentor OpenAIInstrumentor().instrument(tracer_provider=trace_provider) import base64 import httpx from openai import OpenAI client = OpenAI() image_url = "https://upload.wikimedia.org/wikipedia/commons/thumb/d/dd/Gfp-wisconsin-madison-the-nature-boardwalk.jpg/2560px-Gfp-wisconsin-madison-the-nature-boardwalk.jpg" image_media_type = "image/jpeg" image_data = base64.standard_b64encode(httpx.get(image_url).content).decode("utf-8") response = client.chat.completions.create( model="gpt-4o", messages=[ { "role": "user", "content": [ {"type": "text", "text": "What is in this image?"}, { "type": "image_url", "image_url": { "url": "https://upload.wikimedia.org/wikipedia/commons/thumb/d/dd/Gfp-wisconsin-madison-the-nature-boardwalk.jpg/2560px-Gfp-wisconsin-madison-the-nature-boardwalk.jpg", }, }, ], }, ], ) print(response.choices[0].message.content)
Case Study, Blogs and more
Case Study, Blogs and more
Case Study, Blogs and More
Case Study, Blogs and More

Enhancing Meeting Summarization Through Future AGI’s Intelligent Evaluation Framework
50% Increase
Summary Quality
10x Faster
Summary Evaluation

Revolutionizing Lead Generation: How Future AGI Empowers AI SDR Companies with Intelligent Prompt Evaluation
25% Increase
Response rate
10X Faster
Prompt evaluation

Enhancing Meeting Summarization Through Future AGI’s Intelligent Evaluation Framework
50% Increase
Summary Quality
10x Faster
Summary Evaluation

Revolutionizing Lead Generation: How Future AGI Empowers AI SDR Companies with Intelligent Prompt Evaluation
25% Increase
Response rate
10X Faster
Prompt evaluation

Enhancing Meeting Summarization Through Future AGI’s Intelligent Evaluation Framework
50% Increase
Summary Quality
10x Faster
Summary Evaluation

Revolutionizing Lead Generation: How Future AGI Empowers AI SDR Companies with Intelligent Prompt Evaluation
25% Increase
Response rate
10X Faster
Prompt evaluation
Trusted by Developers from around the world
Media Coverage
Media Coverage
Media Coverage
Media Coverage
Feb 11, 2025 | by Forbes
How Future AGI Is Holding AI Applications To AccountFeb 11, 2025 | by The Wall Street Journal
San Francisco based AI application evaluation & optimization platform, raised $1.6M in pre-seed fundingFeb 11, 2025 | by Fortune
A San Francisco based AI evaluation & optimization platform raises $1.6 million in pre-seed fundingFeb 12, 2025 | by Yourstory
AI infrastructure company Future AGI secures $1.6M in pre-seed fundingFeb 11, 2025 | by Axios
An SF based AI infrastructure startup, raises $1.6m in pre-seed co-led by Powerhouse Ventures and Snow leopard venturesFeb 11, 2025 | by Strictly VC
Future AGI announces $1.6M pre-seed round and launches world's most accurate multimodal AI evaluation toolFeb 11, 2025 | by AI Insider
Future AGI announces $1.6 million pre-seed roundFeb 12, 2025 | by Intelligent CIO
Future AGI launches world's most accurate multimodal AI evaluation toolFeb 13, 2025 | by AIM
AI infrastructure startup Future AGI has raised $1.6 million in a pre-seed funding round co-led by Powerhouse Ventures and Snow Leopard Ventures
Feb 11, 2025 | by Forbes
How Future AGI Is Holding AI Applications To AccountFeb 11, 2025 | by The Wall Street Journal
San Francisco based AI application evaluation & optimization platform, raised $1.6M in pre-seed fundingFeb 11, 2025 | by Fortune
A San Francisco based AI evaluation & optimization platform raises $1.6 million in pre-seed fundingFeb 12, 2025 | by Yourstory
AI infrastructure company Future AGI secures $1.6M in pre-seed fundingFeb 11, 2025 | by Axios
An SF based AI infrastructure startup, raises $1.6m in pre-seed co-led by Powerhouse Ventures and Snow leopard venturesFeb 11, 2025 | by Strictly VC
Future AGI announces $1.6M pre-seed round and launches world's most accurate multimodal AI evaluation toolFeb 11, 2025 | by AI Insider
Future AGI announces $1.6 million pre-seed roundFeb 12, 2025 | by Intelligent CIO
Future AGI launches world's most accurate multimodal AI evaluation toolFeb 13, 2025 | by AIM
AI infrastructure startup Future AGI has raised $1.6 million in a pre-seed funding round co-led by Powerhouse Ventures and Snow Leopard Ventures


Ready to deploy Accurate AI?

Ready to deploy Accurate AI?

Ready to deploy Accurate AI?

Ready to deploy Accurate AI?

